Log-likelihood of zero-truncated Poisson
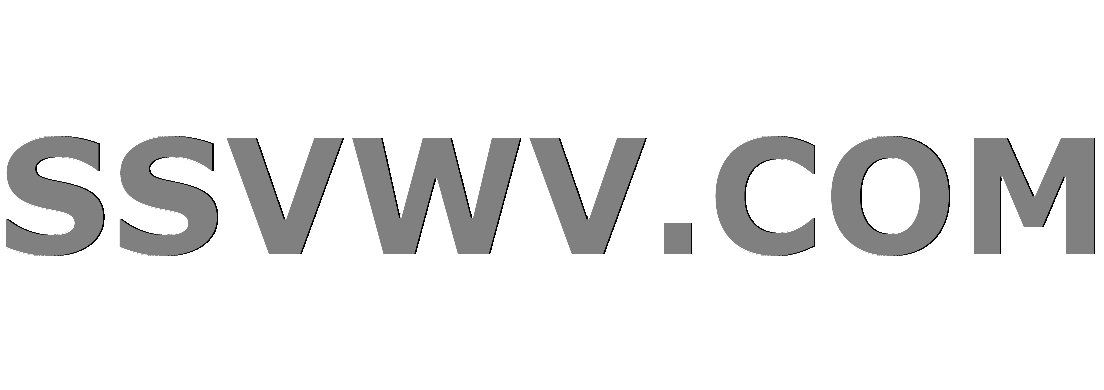
Multi tool use
up vote
1
down vote
favorite
Question:
Edwards and Eberhardt (1967) conducted a live-trapping study on a confined population of known size. In their study, wild cottontail
rabbits were penned in a 4-acre rabbit-proof enclosure. Live trapping was conducted for 18 consecutive
nights. Recorded capture frequencies were as follows: 43 rabbits were caught at exactly 1 night, 16
rabbits at 2 nights, 8 at 3 nights, 6 at four nights, 2 at 6 nights and 1 rabbit was caught at 7 nights. In
other words, we have observations from $X_1, · · · X_m$ count variables of m = 76 different, caught rabbits.
So, in this sample, 43 of the $x_1, · · · , x_m$ were ones, 16 twos, etc. Every count can take a maximum of
T = 18 captures. The number of of zero counts, i.e. the number of rabbits that were not caught, is
unobserved. We denote this additional sample as $X_{m+1}, · · · , X_n$. Assuming independence of trapping
occasions and homogeneity of capture probability θ at each occasion each $X_i$ would follow a binomial distribution. We assume here that a Poisson approximation is justified. That is:
$P(X_i = x) = frac{exp(−θT)(θt)^x}{x!}$ where i = 1, · · · , n.
Note that the observed sample is zero-truncated so that the observed sample can be considered as arising from the zero-truncated Poisson density:
$$exp(−θT)(θT)^x over (1 − exp(−θT))x!$$ for $i = 1, · · · , m$, $;θ ∈ (0, 1)$ and $x ∈ {0, 1, · · · , T}$.
Determine the log-likelihood function for the observed sample.
My solution:
I have already attempted this and got:
$sum_{i=1}^m n_ilogfrac{exp(−θT)(θT)^x}{x!}frac{1}{1 − exp(−θT)}$
Is this correct? If not, what should I have got? What have I done wrong?
poisson-distribution log-likelihood
add a comment |
up vote
1
down vote
favorite
Question:
Edwards and Eberhardt (1967) conducted a live-trapping study on a confined population of known size. In their study, wild cottontail
rabbits were penned in a 4-acre rabbit-proof enclosure. Live trapping was conducted for 18 consecutive
nights. Recorded capture frequencies were as follows: 43 rabbits were caught at exactly 1 night, 16
rabbits at 2 nights, 8 at 3 nights, 6 at four nights, 2 at 6 nights and 1 rabbit was caught at 7 nights. In
other words, we have observations from $X_1, · · · X_m$ count variables of m = 76 different, caught rabbits.
So, in this sample, 43 of the $x_1, · · · , x_m$ were ones, 16 twos, etc. Every count can take a maximum of
T = 18 captures. The number of of zero counts, i.e. the number of rabbits that were not caught, is
unobserved. We denote this additional sample as $X_{m+1}, · · · , X_n$. Assuming independence of trapping
occasions and homogeneity of capture probability θ at each occasion each $X_i$ would follow a binomial distribution. We assume here that a Poisson approximation is justified. That is:
$P(X_i = x) = frac{exp(−θT)(θt)^x}{x!}$ where i = 1, · · · , n.
Note that the observed sample is zero-truncated so that the observed sample can be considered as arising from the zero-truncated Poisson density:
$$exp(−θT)(θT)^x over (1 − exp(−θT))x!$$ for $i = 1, · · · , m$, $;θ ∈ (0, 1)$ and $x ∈ {0, 1, · · · , T}$.
Determine the log-likelihood function for the observed sample.
My solution:
I have already attempted this and got:
$sum_{i=1}^m n_ilogfrac{exp(−θT)(θT)^x}{x!}frac{1}{1 − exp(−θT)}$
Is this correct? If not, what should I have got? What have I done wrong?
poisson-distribution log-likelihood
Someone's been doing the Dankmar's coursework here :P I think the context is not relevant anyway, you can just ask for the log-likelihood function of a zero-truncated Poisson distribution and its first and second order derivative
– Euler_Salter
Nov 21 at 22:42
@Euler_Salter haha yes! I thought context was good. Does it look right to you?
– Vicky
Nov 21 at 22:49
Yes but it could scare off lazy fellow stackexchangers, so it's nice to keep it short! Not sure now, I'm a bit tired, but I just found this: math.stackexchange.com/a/1857529/348937
– Euler_Salter
Nov 21 at 22:51
@Euler_Salter thanks! Will take a look.
– Vicky
Nov 21 at 22:53
add a comment |
up vote
1
down vote
favorite
up vote
1
down vote
favorite
Question:
Edwards and Eberhardt (1967) conducted a live-trapping study on a confined population of known size. In their study, wild cottontail
rabbits were penned in a 4-acre rabbit-proof enclosure. Live trapping was conducted for 18 consecutive
nights. Recorded capture frequencies were as follows: 43 rabbits were caught at exactly 1 night, 16
rabbits at 2 nights, 8 at 3 nights, 6 at four nights, 2 at 6 nights and 1 rabbit was caught at 7 nights. In
other words, we have observations from $X_1, · · · X_m$ count variables of m = 76 different, caught rabbits.
So, in this sample, 43 of the $x_1, · · · , x_m$ were ones, 16 twos, etc. Every count can take a maximum of
T = 18 captures. The number of of zero counts, i.e. the number of rabbits that were not caught, is
unobserved. We denote this additional sample as $X_{m+1}, · · · , X_n$. Assuming independence of trapping
occasions and homogeneity of capture probability θ at each occasion each $X_i$ would follow a binomial distribution. We assume here that a Poisson approximation is justified. That is:
$P(X_i = x) = frac{exp(−θT)(θt)^x}{x!}$ where i = 1, · · · , n.
Note that the observed sample is zero-truncated so that the observed sample can be considered as arising from the zero-truncated Poisson density:
$$exp(−θT)(θT)^x over (1 − exp(−θT))x!$$ for $i = 1, · · · , m$, $;θ ∈ (0, 1)$ and $x ∈ {0, 1, · · · , T}$.
Determine the log-likelihood function for the observed sample.
My solution:
I have already attempted this and got:
$sum_{i=1}^m n_ilogfrac{exp(−θT)(θT)^x}{x!}frac{1}{1 − exp(−θT)}$
Is this correct? If not, what should I have got? What have I done wrong?
poisson-distribution log-likelihood
Question:
Edwards and Eberhardt (1967) conducted a live-trapping study on a confined population of known size. In their study, wild cottontail
rabbits were penned in a 4-acre rabbit-proof enclosure. Live trapping was conducted for 18 consecutive
nights. Recorded capture frequencies were as follows: 43 rabbits were caught at exactly 1 night, 16
rabbits at 2 nights, 8 at 3 nights, 6 at four nights, 2 at 6 nights and 1 rabbit was caught at 7 nights. In
other words, we have observations from $X_1, · · · X_m$ count variables of m = 76 different, caught rabbits.
So, in this sample, 43 of the $x_1, · · · , x_m$ were ones, 16 twos, etc. Every count can take a maximum of
T = 18 captures. The number of of zero counts, i.e. the number of rabbits that were not caught, is
unobserved. We denote this additional sample as $X_{m+1}, · · · , X_n$. Assuming independence of trapping
occasions and homogeneity of capture probability θ at each occasion each $X_i$ would follow a binomial distribution. We assume here that a Poisson approximation is justified. That is:
$P(X_i = x) = frac{exp(−θT)(θt)^x}{x!}$ where i = 1, · · · , n.
Note that the observed sample is zero-truncated so that the observed sample can be considered as arising from the zero-truncated Poisson density:
$$exp(−θT)(θT)^x over (1 − exp(−θT))x!$$ for $i = 1, · · · , m$, $;θ ∈ (0, 1)$ and $x ∈ {0, 1, · · · , T}$.
Determine the log-likelihood function for the observed sample.
My solution:
I have already attempted this and got:
$sum_{i=1}^m n_ilogfrac{exp(−θT)(θT)^x}{x!}frac{1}{1 − exp(−θT)}$
Is this correct? If not, what should I have got? What have I done wrong?
poisson-distribution log-likelihood
poisson-distribution log-likelihood
asked Nov 21 at 22:34
Vicky
597
597
Someone's been doing the Dankmar's coursework here :P I think the context is not relevant anyway, you can just ask for the log-likelihood function of a zero-truncated Poisson distribution and its first and second order derivative
– Euler_Salter
Nov 21 at 22:42
@Euler_Salter haha yes! I thought context was good. Does it look right to you?
– Vicky
Nov 21 at 22:49
Yes but it could scare off lazy fellow stackexchangers, so it's nice to keep it short! Not sure now, I'm a bit tired, but I just found this: math.stackexchange.com/a/1857529/348937
– Euler_Salter
Nov 21 at 22:51
@Euler_Salter thanks! Will take a look.
– Vicky
Nov 21 at 22:53
add a comment |
Someone's been doing the Dankmar's coursework here :P I think the context is not relevant anyway, you can just ask for the log-likelihood function of a zero-truncated Poisson distribution and its first and second order derivative
– Euler_Salter
Nov 21 at 22:42
@Euler_Salter haha yes! I thought context was good. Does it look right to you?
– Vicky
Nov 21 at 22:49
Yes but it could scare off lazy fellow stackexchangers, so it's nice to keep it short! Not sure now, I'm a bit tired, but I just found this: math.stackexchange.com/a/1857529/348937
– Euler_Salter
Nov 21 at 22:51
@Euler_Salter thanks! Will take a look.
– Vicky
Nov 21 at 22:53
Someone's been doing the Dankmar's coursework here :P I think the context is not relevant anyway, you can just ask for the log-likelihood function of a zero-truncated Poisson distribution and its first and second order derivative
– Euler_Salter
Nov 21 at 22:42
Someone's been doing the Dankmar's coursework here :P I think the context is not relevant anyway, you can just ask for the log-likelihood function of a zero-truncated Poisson distribution and its first and second order derivative
– Euler_Salter
Nov 21 at 22:42
@Euler_Salter haha yes! I thought context was good. Does it look right to you?
– Vicky
Nov 21 at 22:49
@Euler_Salter haha yes! I thought context was good. Does it look right to you?
– Vicky
Nov 21 at 22:49
Yes but it could scare off lazy fellow stackexchangers, so it's nice to keep it short! Not sure now, I'm a bit tired, but I just found this: math.stackexchange.com/a/1857529/348937
– Euler_Salter
Nov 21 at 22:51
Yes but it could scare off lazy fellow stackexchangers, so it's nice to keep it short! Not sure now, I'm a bit tired, but I just found this: math.stackexchange.com/a/1857529/348937
– Euler_Salter
Nov 21 at 22:51
@Euler_Salter thanks! Will take a look.
– Vicky
Nov 21 at 22:53
@Euler_Salter thanks! Will take a look.
– Vicky
Nov 21 at 22:53
add a comment |
active
oldest
votes
active
oldest
votes
active
oldest
votes
active
oldest
votes
active
oldest
votes
Sign up or log in
StackExchange.ready(function () {
StackExchange.helpers.onClickDraftSave('#login-link');
});
Sign up using Google
Sign up using Facebook
Sign up using Email and Password
Post as a guest
Required, but never shown
StackExchange.ready(
function () {
StackExchange.openid.initPostLogin('.new-post-login', 'https%3a%2f%2fmath.stackexchange.com%2fquestions%2f3008478%2flog-likelihood-of-zero-truncated-poisson%23new-answer', 'question_page');
}
);
Post as a guest
Required, but never shown
Sign up or log in
StackExchange.ready(function () {
StackExchange.helpers.onClickDraftSave('#login-link');
});
Sign up using Google
Sign up using Facebook
Sign up using Email and Password
Post as a guest
Required, but never shown
Sign up or log in
StackExchange.ready(function () {
StackExchange.helpers.onClickDraftSave('#login-link');
});
Sign up using Google
Sign up using Facebook
Sign up using Email and Password
Post as a guest
Required, but never shown
Sign up or log in
StackExchange.ready(function () {
StackExchange.helpers.onClickDraftSave('#login-link');
});
Sign up using Google
Sign up using Facebook
Sign up using Email and Password
Sign up using Google
Sign up using Facebook
Sign up using Email and Password
Post as a guest
Required, but never shown
Required, but never shown
Required, but never shown
Required, but never shown
Required, but never shown
Required, but never shown
Required, but never shown
Required, but never shown
Required, but never shown
ocGe2cPK,8,IzdpgZU HuzMQ8Wof4I2x7I30,ohd,qbNPuMruRy85KPGtQW,jh,Q zy0j6pf865Vv60nL6,M
Someone's been doing the Dankmar's coursework here :P I think the context is not relevant anyway, you can just ask for the log-likelihood function of a zero-truncated Poisson distribution and its first and second order derivative
– Euler_Salter
Nov 21 at 22:42
@Euler_Salter haha yes! I thought context was good. Does it look right to you?
– Vicky
Nov 21 at 22:49
Yes but it could scare off lazy fellow stackexchangers, so it's nice to keep it short! Not sure now, I'm a bit tired, but I just found this: math.stackexchange.com/a/1857529/348937
– Euler_Salter
Nov 21 at 22:51
@Euler_Salter thanks! Will take a look.
– Vicky
Nov 21 at 22:53