Marginal Density Function, Gamma and Beta distributions
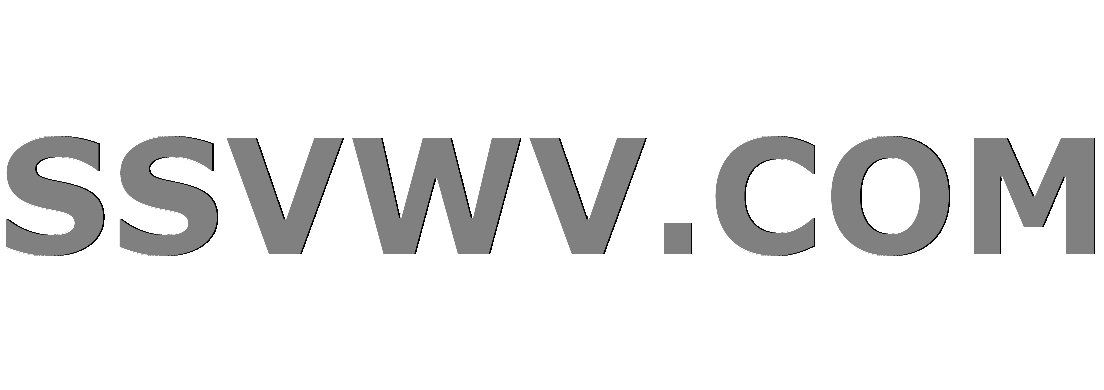
Multi tool use
If $Ysimoperatorname{Gamma}(gamma,delta)$ and $Zsimoperatorname{Beta}(alpha,beta)$ then their density functions are, respectively,
$$
f_Y(y)=frac{delta^gamma}{Gamma(gamma)}y^{gamma-1}e^{-delta y},quad y>0,quadgamma>0,quaddelta>0
$$
and
$$
f_Z(z)=frac{Gamma(alpha+beta)}{Gamma(alpha)Gamma(beta)}z^{alpha-1}(1-z)^{beta-1},quad 0leq zleq 1,quadalpha>0,quadbeta>0.
$$
Consider $X_1$ and $X_2$ having $operatorname{Gamma}(a+b,1)$ and $operatorname{Beta}(a,b)$ distributions, respectively, where $a,b>0$. Assume that $X_1$ and $X_2$ are independent.
How do i find the marginal density functions of $Y_1 = X_1X_2$ and $Y_2 = X_1(1-X_2)$?
I know that the marginal density function can be derived from the joint density, but since the joint is not given, how do I create it?
Also how do I manipulate the gamma function? first time I have come across it.
probability probability-theory probability-distributions density-function
add a comment |
If $Ysimoperatorname{Gamma}(gamma,delta)$ and $Zsimoperatorname{Beta}(alpha,beta)$ then their density functions are, respectively,
$$
f_Y(y)=frac{delta^gamma}{Gamma(gamma)}y^{gamma-1}e^{-delta y},quad y>0,quadgamma>0,quaddelta>0
$$
and
$$
f_Z(z)=frac{Gamma(alpha+beta)}{Gamma(alpha)Gamma(beta)}z^{alpha-1}(1-z)^{beta-1},quad 0leq zleq 1,quadalpha>0,quadbeta>0.
$$
Consider $X_1$ and $X_2$ having $operatorname{Gamma}(a+b,1)$ and $operatorname{Beta}(a,b)$ distributions, respectively, where $a,b>0$. Assume that $X_1$ and $X_2$ are independent.
How do i find the marginal density functions of $Y_1 = X_1X_2$ and $Y_2 = X_1(1-X_2)$?
I know that the marginal density function can be derived from the joint density, but since the joint is not given, how do I create it?
Also how do I manipulate the gamma function? first time I have come across it.
probability probability-theory probability-distributions density-function
3
Perhaps you meant how to find the joint density of $(Y_1,Y_2)$. Do you know change of variables?
– StubbornAtom
Dec 1 at 10:55
@StubbornAtom thank you for your response. By change of variables you mean for integration?
– OvermanZarathustra
Dec 4 at 0:00
add a comment |
If $Ysimoperatorname{Gamma}(gamma,delta)$ and $Zsimoperatorname{Beta}(alpha,beta)$ then their density functions are, respectively,
$$
f_Y(y)=frac{delta^gamma}{Gamma(gamma)}y^{gamma-1}e^{-delta y},quad y>0,quadgamma>0,quaddelta>0
$$
and
$$
f_Z(z)=frac{Gamma(alpha+beta)}{Gamma(alpha)Gamma(beta)}z^{alpha-1}(1-z)^{beta-1},quad 0leq zleq 1,quadalpha>0,quadbeta>0.
$$
Consider $X_1$ and $X_2$ having $operatorname{Gamma}(a+b,1)$ and $operatorname{Beta}(a,b)$ distributions, respectively, where $a,b>0$. Assume that $X_1$ and $X_2$ are independent.
How do i find the marginal density functions of $Y_1 = X_1X_2$ and $Y_2 = X_1(1-X_2)$?
I know that the marginal density function can be derived from the joint density, but since the joint is not given, how do I create it?
Also how do I manipulate the gamma function? first time I have come across it.
probability probability-theory probability-distributions density-function
If $Ysimoperatorname{Gamma}(gamma,delta)$ and $Zsimoperatorname{Beta}(alpha,beta)$ then their density functions are, respectively,
$$
f_Y(y)=frac{delta^gamma}{Gamma(gamma)}y^{gamma-1}e^{-delta y},quad y>0,quadgamma>0,quaddelta>0
$$
and
$$
f_Z(z)=frac{Gamma(alpha+beta)}{Gamma(alpha)Gamma(beta)}z^{alpha-1}(1-z)^{beta-1},quad 0leq zleq 1,quadalpha>0,quadbeta>0.
$$
Consider $X_1$ and $X_2$ having $operatorname{Gamma}(a+b,1)$ and $operatorname{Beta}(a,b)$ distributions, respectively, where $a,b>0$. Assume that $X_1$ and $X_2$ are independent.
How do i find the marginal density functions of $Y_1 = X_1X_2$ and $Y_2 = X_1(1-X_2)$?
I know that the marginal density function can be derived from the joint density, but since the joint is not given, how do I create it?
Also how do I manipulate the gamma function? first time I have come across it.
probability probability-theory probability-distributions density-function
probability probability-theory probability-distributions density-function
edited Dec 1 at 11:57
user10354138
7,3592824
7,3592824
asked Dec 1 at 10:44
OvermanZarathustra
156
156
3
Perhaps you meant how to find the joint density of $(Y_1,Y_2)$. Do you know change of variables?
– StubbornAtom
Dec 1 at 10:55
@StubbornAtom thank you for your response. By change of variables you mean for integration?
– OvermanZarathustra
Dec 4 at 0:00
add a comment |
3
Perhaps you meant how to find the joint density of $(Y_1,Y_2)$. Do you know change of variables?
– StubbornAtom
Dec 1 at 10:55
@StubbornAtom thank you for your response. By change of variables you mean for integration?
– OvermanZarathustra
Dec 4 at 0:00
3
3
Perhaps you meant how to find the joint density of $(Y_1,Y_2)$. Do you know change of variables?
– StubbornAtom
Dec 1 at 10:55
Perhaps you meant how to find the joint density of $(Y_1,Y_2)$. Do you know change of variables?
– StubbornAtom
Dec 1 at 10:55
@StubbornAtom thank you for your response. By change of variables you mean for integration?
– OvermanZarathustra
Dec 4 at 0:00
@StubbornAtom thank you for your response. By change of variables you mean for integration?
– OvermanZarathustra
Dec 4 at 0:00
add a comment |
1 Answer
1
active
oldest
votes
Plugging in the definition, $X_1$ following $operatorname{Gamma}(a+b,1)$ means its density is
$$f_{X_1}(x_1) = frac1{ Gamma(a+b)}, x_1^{a+b-1} e^{-x_1} qquad text{for}~~ 0 < x_1 < infty $$
The density of $X_2$ is
$$f_{X_2}(x_2) = frac{ Gamma(a+b) }{ Gamma(a) Gamma(b) }, x_2^{a-1} (1 - x_2)^{b-1} qquad text{for}~~ 0<x_2<1$$
The fact that $X_1 perp X_2$ means their joint density is just the direct product
$$f_{X_1X_2}(x_1,, x_2) = frac1{ Gamma(a) Gamma(b) }, x_1^{a+b-1} e^{-x_1} , x_2^{a-1} (1 - x_2)^{b-1} qquad text{for}~~ begin{cases}
0<x_1<infty \
0<x_2<1 end{cases} tag*{Eq.(1)}$$
The 2-dim transformation is
$$begin{cases}
Y_1 = X_1 X_2 \
\
Y_2 = X_1 (1 - X_2)
end{cases} Longleftrightarrow begin{cases}
X_1 = Y_1 + Y_2 \
\
X_2 = dfrac{ Y_1 }{ Y_1 + Y_2}
end{cases} qquad text{where}~~ begin{cases}
0<y_1<infty \
0<y_2<infty end{cases}$$
with the Jacobian (of the inverse mapping) as
$$J = left| begin{matrix} dfrac{ partial x_1}{ partial y_1} & dfrac{ partial x_1}{ partial y_2} \
dfrac{ partial x_2}{ partial y_1} & dfrac{ partial x_2}{ partial y_2}end{matrix} right| = left| begin{matrix} 1 & 1 \
dfrac{ y_2 }{ (y_1 +y_2)^2 } & dfrac{ -y_1 }{ (y_1 +y_2)^2 } end{matrix} right| = frac{-1}{ y_1 + y_2 }$$
The transformed joint density for $Y_1$ and $Y_2$ is
begin{align}
f_{Y_1Y_2}( y_1 ,~y_2 ) &= |J| cdot f_{X_1X_2}( x_1,, x_2)Bigg|_{x_1 = y_1+y_2, ,,x_2 = frac{y_1}{y_1 + y_2}} qquad text{, plug in Eq.(1)}\
&= frac1{ y_1 + y_2} cdot frac1{ Gamma(a) Gamma(b) }, (y_1 + y_2)^{a+b-1} e^{-(y_1 + y_2)} , left(frac{y_1}{ y_1 + y_2}right)^{a-1} left(frac{y_2}{ y_1 + y_2} right)^{b-1} \
&= frac1{ Gamma(a) Gamma(b) }, y_1^{a-1} y_2^{b-1} e^{-(y_1 + y_2)} qquad text{for}~~0<y_1<infty ,~0<y_2<infty
end{align}
The marginal density of $Y_1$ can be obtained from the joint as
begin{align}
f_{Y_1}(y_1) &= int_{y_2 = 0}^{infty} f_{Y_1Y_2}( y_1 ,~y_2 ) ,mathrm{d}y_2 \
&= frac1{Gamma(a)} y_1^{a-1} e^{-y_1} int_{y_2 = 0}^{infty} frac1{Gamma(b)} y_2^{b-1} e^{-y_2} ,mathrm{d}y_2 qquad scriptsizetext{integral is just the kernel of Gamma distribution} \
&= frac1{Gamma(a)} y_1^{a-1} e^{-y_1}
end{align}
Thus one identifies the distribution of $Y_1$ as $operatorname{Gamma}(a,1)$.
Similarly, or noting the symmetry in the joint $f_{Y_1Y_2}( y_1 ,~y_2 )$, we have $Y_2$ follows $operatorname{Gamma}(b,1)$.
Thank you very much for your response! Is there another way to solve this question? I don't think i understand the Jacobian method.
– OvermanZarathustra
Dec 3 at 18:23
1
It is a well-known basic fact that Beta distribution can be viewed as $frac{U}{U+W}$ where $U$ and $W$ are independent and follow Gamma distributions (of the same rate/scale parameter). See this or the 10th item of this. The question is just a symbolic (verbal) argument of the inverse of this definition. However, this way of quoting "known results" is hardly what the question seems to be aiming for.
– Lee David Chung Lin
Dec 4 at 11:01
1
The question statement basically gives you the joint $f_{X1X2}(x_1,x_2)$ and the intention is most likely asking you to do some calculus one way or the other. For example, you can do the CDF of $Y_1$ as $Pr{Y_1 < y_1 } = Pr{X_1 X_2 < y_1 }$, which is a 2-dim integration across the relevant region (bounded by the hyperbola $x_1 x_2 = y_1$ and $x_2 = 0$, $x_2 = 1$, along with $x_1 = 0$) over the joint density $f_{X_1X_2}(x_1,x_2)$. I doubt you'll find this easier, but if you'd like to see it I can make another answer post.
– Lee David Chung Lin
Dec 4 at 11:06
1
Meanwhile, originally you posted the question with a snapshot. Which textbook is it? It's rather unlikely that the method of variable transformation (1-dim and 2-dim) is not covered, even if it's only a half-decent material.
– Lee David Chung Lin
Dec 4 at 11:38
add a comment |
Your Answer
StackExchange.ifUsing("editor", function () {
return StackExchange.using("mathjaxEditing", function () {
StackExchange.MarkdownEditor.creationCallbacks.add(function (editor, postfix) {
StackExchange.mathjaxEditing.prepareWmdForMathJax(editor, postfix, [["$", "$"], ["\\(","\\)"]]);
});
});
}, "mathjax-editing");
StackExchange.ready(function() {
var channelOptions = {
tags: "".split(" "),
id: "69"
};
initTagRenderer("".split(" "), "".split(" "), channelOptions);
StackExchange.using("externalEditor", function() {
// Have to fire editor after snippets, if snippets enabled
if (StackExchange.settings.snippets.snippetsEnabled) {
StackExchange.using("snippets", function() {
createEditor();
});
}
else {
createEditor();
}
});
function createEditor() {
StackExchange.prepareEditor({
heartbeatType: 'answer',
autoActivateHeartbeat: false,
convertImagesToLinks: true,
noModals: true,
showLowRepImageUploadWarning: true,
reputationToPostImages: 10,
bindNavPrevention: true,
postfix: "",
imageUploader: {
brandingHtml: "Powered by u003ca class="icon-imgur-white" href="https://imgur.com/"u003eu003c/au003e",
contentPolicyHtml: "User contributions licensed under u003ca href="https://creativecommons.org/licenses/by-sa/3.0/"u003ecc by-sa 3.0 with attribution requiredu003c/au003e u003ca href="https://stackoverflow.com/legal/content-policy"u003e(content policy)u003c/au003e",
allowUrls: true
},
noCode: true, onDemand: true,
discardSelector: ".discard-answer"
,immediatelyShowMarkdownHelp:true
});
}
});
Sign up or log in
StackExchange.ready(function () {
StackExchange.helpers.onClickDraftSave('#login-link');
});
Sign up using Google
Sign up using Facebook
Sign up using Email and Password
Post as a guest
Required, but never shown
StackExchange.ready(
function () {
StackExchange.openid.initPostLogin('.new-post-login', 'https%3a%2f%2fmath.stackexchange.com%2fquestions%2f3021216%2fmarginal-density-function-gamma-and-beta-distributions%23new-answer', 'question_page');
}
);
Post as a guest
Required, but never shown
1 Answer
1
active
oldest
votes
1 Answer
1
active
oldest
votes
active
oldest
votes
active
oldest
votes
Plugging in the definition, $X_1$ following $operatorname{Gamma}(a+b,1)$ means its density is
$$f_{X_1}(x_1) = frac1{ Gamma(a+b)}, x_1^{a+b-1} e^{-x_1} qquad text{for}~~ 0 < x_1 < infty $$
The density of $X_2$ is
$$f_{X_2}(x_2) = frac{ Gamma(a+b) }{ Gamma(a) Gamma(b) }, x_2^{a-1} (1 - x_2)^{b-1} qquad text{for}~~ 0<x_2<1$$
The fact that $X_1 perp X_2$ means their joint density is just the direct product
$$f_{X_1X_2}(x_1,, x_2) = frac1{ Gamma(a) Gamma(b) }, x_1^{a+b-1} e^{-x_1} , x_2^{a-1} (1 - x_2)^{b-1} qquad text{for}~~ begin{cases}
0<x_1<infty \
0<x_2<1 end{cases} tag*{Eq.(1)}$$
The 2-dim transformation is
$$begin{cases}
Y_1 = X_1 X_2 \
\
Y_2 = X_1 (1 - X_2)
end{cases} Longleftrightarrow begin{cases}
X_1 = Y_1 + Y_2 \
\
X_2 = dfrac{ Y_1 }{ Y_1 + Y_2}
end{cases} qquad text{where}~~ begin{cases}
0<y_1<infty \
0<y_2<infty end{cases}$$
with the Jacobian (of the inverse mapping) as
$$J = left| begin{matrix} dfrac{ partial x_1}{ partial y_1} & dfrac{ partial x_1}{ partial y_2} \
dfrac{ partial x_2}{ partial y_1} & dfrac{ partial x_2}{ partial y_2}end{matrix} right| = left| begin{matrix} 1 & 1 \
dfrac{ y_2 }{ (y_1 +y_2)^2 } & dfrac{ -y_1 }{ (y_1 +y_2)^2 } end{matrix} right| = frac{-1}{ y_1 + y_2 }$$
The transformed joint density for $Y_1$ and $Y_2$ is
begin{align}
f_{Y_1Y_2}( y_1 ,~y_2 ) &= |J| cdot f_{X_1X_2}( x_1,, x_2)Bigg|_{x_1 = y_1+y_2, ,,x_2 = frac{y_1}{y_1 + y_2}} qquad text{, plug in Eq.(1)}\
&= frac1{ y_1 + y_2} cdot frac1{ Gamma(a) Gamma(b) }, (y_1 + y_2)^{a+b-1} e^{-(y_1 + y_2)} , left(frac{y_1}{ y_1 + y_2}right)^{a-1} left(frac{y_2}{ y_1 + y_2} right)^{b-1} \
&= frac1{ Gamma(a) Gamma(b) }, y_1^{a-1} y_2^{b-1} e^{-(y_1 + y_2)} qquad text{for}~~0<y_1<infty ,~0<y_2<infty
end{align}
The marginal density of $Y_1$ can be obtained from the joint as
begin{align}
f_{Y_1}(y_1) &= int_{y_2 = 0}^{infty} f_{Y_1Y_2}( y_1 ,~y_2 ) ,mathrm{d}y_2 \
&= frac1{Gamma(a)} y_1^{a-1} e^{-y_1} int_{y_2 = 0}^{infty} frac1{Gamma(b)} y_2^{b-1} e^{-y_2} ,mathrm{d}y_2 qquad scriptsizetext{integral is just the kernel of Gamma distribution} \
&= frac1{Gamma(a)} y_1^{a-1} e^{-y_1}
end{align}
Thus one identifies the distribution of $Y_1$ as $operatorname{Gamma}(a,1)$.
Similarly, or noting the symmetry in the joint $f_{Y_1Y_2}( y_1 ,~y_2 )$, we have $Y_2$ follows $operatorname{Gamma}(b,1)$.
Thank you very much for your response! Is there another way to solve this question? I don't think i understand the Jacobian method.
– OvermanZarathustra
Dec 3 at 18:23
1
It is a well-known basic fact that Beta distribution can be viewed as $frac{U}{U+W}$ where $U$ and $W$ are independent and follow Gamma distributions (of the same rate/scale parameter). See this or the 10th item of this. The question is just a symbolic (verbal) argument of the inverse of this definition. However, this way of quoting "known results" is hardly what the question seems to be aiming for.
– Lee David Chung Lin
Dec 4 at 11:01
1
The question statement basically gives you the joint $f_{X1X2}(x_1,x_2)$ and the intention is most likely asking you to do some calculus one way or the other. For example, you can do the CDF of $Y_1$ as $Pr{Y_1 < y_1 } = Pr{X_1 X_2 < y_1 }$, which is a 2-dim integration across the relevant region (bounded by the hyperbola $x_1 x_2 = y_1$ and $x_2 = 0$, $x_2 = 1$, along with $x_1 = 0$) over the joint density $f_{X_1X_2}(x_1,x_2)$. I doubt you'll find this easier, but if you'd like to see it I can make another answer post.
– Lee David Chung Lin
Dec 4 at 11:06
1
Meanwhile, originally you posted the question with a snapshot. Which textbook is it? It's rather unlikely that the method of variable transformation (1-dim and 2-dim) is not covered, even if it's only a half-decent material.
– Lee David Chung Lin
Dec 4 at 11:38
add a comment |
Plugging in the definition, $X_1$ following $operatorname{Gamma}(a+b,1)$ means its density is
$$f_{X_1}(x_1) = frac1{ Gamma(a+b)}, x_1^{a+b-1} e^{-x_1} qquad text{for}~~ 0 < x_1 < infty $$
The density of $X_2$ is
$$f_{X_2}(x_2) = frac{ Gamma(a+b) }{ Gamma(a) Gamma(b) }, x_2^{a-1} (1 - x_2)^{b-1} qquad text{for}~~ 0<x_2<1$$
The fact that $X_1 perp X_2$ means their joint density is just the direct product
$$f_{X_1X_2}(x_1,, x_2) = frac1{ Gamma(a) Gamma(b) }, x_1^{a+b-1} e^{-x_1} , x_2^{a-1} (1 - x_2)^{b-1} qquad text{for}~~ begin{cases}
0<x_1<infty \
0<x_2<1 end{cases} tag*{Eq.(1)}$$
The 2-dim transformation is
$$begin{cases}
Y_1 = X_1 X_2 \
\
Y_2 = X_1 (1 - X_2)
end{cases} Longleftrightarrow begin{cases}
X_1 = Y_1 + Y_2 \
\
X_2 = dfrac{ Y_1 }{ Y_1 + Y_2}
end{cases} qquad text{where}~~ begin{cases}
0<y_1<infty \
0<y_2<infty end{cases}$$
with the Jacobian (of the inverse mapping) as
$$J = left| begin{matrix} dfrac{ partial x_1}{ partial y_1} & dfrac{ partial x_1}{ partial y_2} \
dfrac{ partial x_2}{ partial y_1} & dfrac{ partial x_2}{ partial y_2}end{matrix} right| = left| begin{matrix} 1 & 1 \
dfrac{ y_2 }{ (y_1 +y_2)^2 } & dfrac{ -y_1 }{ (y_1 +y_2)^2 } end{matrix} right| = frac{-1}{ y_1 + y_2 }$$
The transformed joint density for $Y_1$ and $Y_2$ is
begin{align}
f_{Y_1Y_2}( y_1 ,~y_2 ) &= |J| cdot f_{X_1X_2}( x_1,, x_2)Bigg|_{x_1 = y_1+y_2, ,,x_2 = frac{y_1}{y_1 + y_2}} qquad text{, plug in Eq.(1)}\
&= frac1{ y_1 + y_2} cdot frac1{ Gamma(a) Gamma(b) }, (y_1 + y_2)^{a+b-1} e^{-(y_1 + y_2)} , left(frac{y_1}{ y_1 + y_2}right)^{a-1} left(frac{y_2}{ y_1 + y_2} right)^{b-1} \
&= frac1{ Gamma(a) Gamma(b) }, y_1^{a-1} y_2^{b-1} e^{-(y_1 + y_2)} qquad text{for}~~0<y_1<infty ,~0<y_2<infty
end{align}
The marginal density of $Y_1$ can be obtained from the joint as
begin{align}
f_{Y_1}(y_1) &= int_{y_2 = 0}^{infty} f_{Y_1Y_2}( y_1 ,~y_2 ) ,mathrm{d}y_2 \
&= frac1{Gamma(a)} y_1^{a-1} e^{-y_1} int_{y_2 = 0}^{infty} frac1{Gamma(b)} y_2^{b-1} e^{-y_2} ,mathrm{d}y_2 qquad scriptsizetext{integral is just the kernel of Gamma distribution} \
&= frac1{Gamma(a)} y_1^{a-1} e^{-y_1}
end{align}
Thus one identifies the distribution of $Y_1$ as $operatorname{Gamma}(a,1)$.
Similarly, or noting the symmetry in the joint $f_{Y_1Y_2}( y_1 ,~y_2 )$, we have $Y_2$ follows $operatorname{Gamma}(b,1)$.
Thank you very much for your response! Is there another way to solve this question? I don't think i understand the Jacobian method.
– OvermanZarathustra
Dec 3 at 18:23
1
It is a well-known basic fact that Beta distribution can be viewed as $frac{U}{U+W}$ where $U$ and $W$ are independent and follow Gamma distributions (of the same rate/scale parameter). See this or the 10th item of this. The question is just a symbolic (verbal) argument of the inverse of this definition. However, this way of quoting "known results" is hardly what the question seems to be aiming for.
– Lee David Chung Lin
Dec 4 at 11:01
1
The question statement basically gives you the joint $f_{X1X2}(x_1,x_2)$ and the intention is most likely asking you to do some calculus one way or the other. For example, you can do the CDF of $Y_1$ as $Pr{Y_1 < y_1 } = Pr{X_1 X_2 < y_1 }$, which is a 2-dim integration across the relevant region (bounded by the hyperbola $x_1 x_2 = y_1$ and $x_2 = 0$, $x_2 = 1$, along with $x_1 = 0$) over the joint density $f_{X_1X_2}(x_1,x_2)$. I doubt you'll find this easier, but if you'd like to see it I can make another answer post.
– Lee David Chung Lin
Dec 4 at 11:06
1
Meanwhile, originally you posted the question with a snapshot. Which textbook is it? It's rather unlikely that the method of variable transformation (1-dim and 2-dim) is not covered, even if it's only a half-decent material.
– Lee David Chung Lin
Dec 4 at 11:38
add a comment |
Plugging in the definition, $X_1$ following $operatorname{Gamma}(a+b,1)$ means its density is
$$f_{X_1}(x_1) = frac1{ Gamma(a+b)}, x_1^{a+b-1} e^{-x_1} qquad text{for}~~ 0 < x_1 < infty $$
The density of $X_2$ is
$$f_{X_2}(x_2) = frac{ Gamma(a+b) }{ Gamma(a) Gamma(b) }, x_2^{a-1} (1 - x_2)^{b-1} qquad text{for}~~ 0<x_2<1$$
The fact that $X_1 perp X_2$ means their joint density is just the direct product
$$f_{X_1X_2}(x_1,, x_2) = frac1{ Gamma(a) Gamma(b) }, x_1^{a+b-1} e^{-x_1} , x_2^{a-1} (1 - x_2)^{b-1} qquad text{for}~~ begin{cases}
0<x_1<infty \
0<x_2<1 end{cases} tag*{Eq.(1)}$$
The 2-dim transformation is
$$begin{cases}
Y_1 = X_1 X_2 \
\
Y_2 = X_1 (1 - X_2)
end{cases} Longleftrightarrow begin{cases}
X_1 = Y_1 + Y_2 \
\
X_2 = dfrac{ Y_1 }{ Y_1 + Y_2}
end{cases} qquad text{where}~~ begin{cases}
0<y_1<infty \
0<y_2<infty end{cases}$$
with the Jacobian (of the inverse mapping) as
$$J = left| begin{matrix} dfrac{ partial x_1}{ partial y_1} & dfrac{ partial x_1}{ partial y_2} \
dfrac{ partial x_2}{ partial y_1} & dfrac{ partial x_2}{ partial y_2}end{matrix} right| = left| begin{matrix} 1 & 1 \
dfrac{ y_2 }{ (y_1 +y_2)^2 } & dfrac{ -y_1 }{ (y_1 +y_2)^2 } end{matrix} right| = frac{-1}{ y_1 + y_2 }$$
The transformed joint density for $Y_1$ and $Y_2$ is
begin{align}
f_{Y_1Y_2}( y_1 ,~y_2 ) &= |J| cdot f_{X_1X_2}( x_1,, x_2)Bigg|_{x_1 = y_1+y_2, ,,x_2 = frac{y_1}{y_1 + y_2}} qquad text{, plug in Eq.(1)}\
&= frac1{ y_1 + y_2} cdot frac1{ Gamma(a) Gamma(b) }, (y_1 + y_2)^{a+b-1} e^{-(y_1 + y_2)} , left(frac{y_1}{ y_1 + y_2}right)^{a-1} left(frac{y_2}{ y_1 + y_2} right)^{b-1} \
&= frac1{ Gamma(a) Gamma(b) }, y_1^{a-1} y_2^{b-1} e^{-(y_1 + y_2)} qquad text{for}~~0<y_1<infty ,~0<y_2<infty
end{align}
The marginal density of $Y_1$ can be obtained from the joint as
begin{align}
f_{Y_1}(y_1) &= int_{y_2 = 0}^{infty} f_{Y_1Y_2}( y_1 ,~y_2 ) ,mathrm{d}y_2 \
&= frac1{Gamma(a)} y_1^{a-1} e^{-y_1} int_{y_2 = 0}^{infty} frac1{Gamma(b)} y_2^{b-1} e^{-y_2} ,mathrm{d}y_2 qquad scriptsizetext{integral is just the kernel of Gamma distribution} \
&= frac1{Gamma(a)} y_1^{a-1} e^{-y_1}
end{align}
Thus one identifies the distribution of $Y_1$ as $operatorname{Gamma}(a,1)$.
Similarly, or noting the symmetry in the joint $f_{Y_1Y_2}( y_1 ,~y_2 )$, we have $Y_2$ follows $operatorname{Gamma}(b,1)$.
Plugging in the definition, $X_1$ following $operatorname{Gamma}(a+b,1)$ means its density is
$$f_{X_1}(x_1) = frac1{ Gamma(a+b)}, x_1^{a+b-1} e^{-x_1} qquad text{for}~~ 0 < x_1 < infty $$
The density of $X_2$ is
$$f_{X_2}(x_2) = frac{ Gamma(a+b) }{ Gamma(a) Gamma(b) }, x_2^{a-1} (1 - x_2)^{b-1} qquad text{for}~~ 0<x_2<1$$
The fact that $X_1 perp X_2$ means their joint density is just the direct product
$$f_{X_1X_2}(x_1,, x_2) = frac1{ Gamma(a) Gamma(b) }, x_1^{a+b-1} e^{-x_1} , x_2^{a-1} (1 - x_2)^{b-1} qquad text{for}~~ begin{cases}
0<x_1<infty \
0<x_2<1 end{cases} tag*{Eq.(1)}$$
The 2-dim transformation is
$$begin{cases}
Y_1 = X_1 X_2 \
\
Y_2 = X_1 (1 - X_2)
end{cases} Longleftrightarrow begin{cases}
X_1 = Y_1 + Y_2 \
\
X_2 = dfrac{ Y_1 }{ Y_1 + Y_2}
end{cases} qquad text{where}~~ begin{cases}
0<y_1<infty \
0<y_2<infty end{cases}$$
with the Jacobian (of the inverse mapping) as
$$J = left| begin{matrix} dfrac{ partial x_1}{ partial y_1} & dfrac{ partial x_1}{ partial y_2} \
dfrac{ partial x_2}{ partial y_1} & dfrac{ partial x_2}{ partial y_2}end{matrix} right| = left| begin{matrix} 1 & 1 \
dfrac{ y_2 }{ (y_1 +y_2)^2 } & dfrac{ -y_1 }{ (y_1 +y_2)^2 } end{matrix} right| = frac{-1}{ y_1 + y_2 }$$
The transformed joint density for $Y_1$ and $Y_2$ is
begin{align}
f_{Y_1Y_2}( y_1 ,~y_2 ) &= |J| cdot f_{X_1X_2}( x_1,, x_2)Bigg|_{x_1 = y_1+y_2, ,,x_2 = frac{y_1}{y_1 + y_2}} qquad text{, plug in Eq.(1)}\
&= frac1{ y_1 + y_2} cdot frac1{ Gamma(a) Gamma(b) }, (y_1 + y_2)^{a+b-1} e^{-(y_1 + y_2)} , left(frac{y_1}{ y_1 + y_2}right)^{a-1} left(frac{y_2}{ y_1 + y_2} right)^{b-1} \
&= frac1{ Gamma(a) Gamma(b) }, y_1^{a-1} y_2^{b-1} e^{-(y_1 + y_2)} qquad text{for}~~0<y_1<infty ,~0<y_2<infty
end{align}
The marginal density of $Y_1$ can be obtained from the joint as
begin{align}
f_{Y_1}(y_1) &= int_{y_2 = 0}^{infty} f_{Y_1Y_2}( y_1 ,~y_2 ) ,mathrm{d}y_2 \
&= frac1{Gamma(a)} y_1^{a-1} e^{-y_1} int_{y_2 = 0}^{infty} frac1{Gamma(b)} y_2^{b-1} e^{-y_2} ,mathrm{d}y_2 qquad scriptsizetext{integral is just the kernel of Gamma distribution} \
&= frac1{Gamma(a)} y_1^{a-1} e^{-y_1}
end{align}
Thus one identifies the distribution of $Y_1$ as $operatorname{Gamma}(a,1)$.
Similarly, or noting the symmetry in the joint $f_{Y_1Y_2}( y_1 ,~y_2 )$, we have $Y_2$ follows $operatorname{Gamma}(b,1)$.
answered Dec 1 at 15:17


Lee David Chung Lin
3,57531039
3,57531039
Thank you very much for your response! Is there another way to solve this question? I don't think i understand the Jacobian method.
– OvermanZarathustra
Dec 3 at 18:23
1
It is a well-known basic fact that Beta distribution can be viewed as $frac{U}{U+W}$ where $U$ and $W$ are independent and follow Gamma distributions (of the same rate/scale parameter). See this or the 10th item of this. The question is just a symbolic (verbal) argument of the inverse of this definition. However, this way of quoting "known results" is hardly what the question seems to be aiming for.
– Lee David Chung Lin
Dec 4 at 11:01
1
The question statement basically gives you the joint $f_{X1X2}(x_1,x_2)$ and the intention is most likely asking you to do some calculus one way or the other. For example, you can do the CDF of $Y_1$ as $Pr{Y_1 < y_1 } = Pr{X_1 X_2 < y_1 }$, which is a 2-dim integration across the relevant region (bounded by the hyperbola $x_1 x_2 = y_1$ and $x_2 = 0$, $x_2 = 1$, along with $x_1 = 0$) over the joint density $f_{X_1X_2}(x_1,x_2)$. I doubt you'll find this easier, but if you'd like to see it I can make another answer post.
– Lee David Chung Lin
Dec 4 at 11:06
1
Meanwhile, originally you posted the question with a snapshot. Which textbook is it? It's rather unlikely that the method of variable transformation (1-dim and 2-dim) is not covered, even if it's only a half-decent material.
– Lee David Chung Lin
Dec 4 at 11:38
add a comment |
Thank you very much for your response! Is there another way to solve this question? I don't think i understand the Jacobian method.
– OvermanZarathustra
Dec 3 at 18:23
1
It is a well-known basic fact that Beta distribution can be viewed as $frac{U}{U+W}$ where $U$ and $W$ are independent and follow Gamma distributions (of the same rate/scale parameter). See this or the 10th item of this. The question is just a symbolic (verbal) argument of the inverse of this definition. However, this way of quoting "known results" is hardly what the question seems to be aiming for.
– Lee David Chung Lin
Dec 4 at 11:01
1
The question statement basically gives you the joint $f_{X1X2}(x_1,x_2)$ and the intention is most likely asking you to do some calculus one way or the other. For example, you can do the CDF of $Y_1$ as $Pr{Y_1 < y_1 } = Pr{X_1 X_2 < y_1 }$, which is a 2-dim integration across the relevant region (bounded by the hyperbola $x_1 x_2 = y_1$ and $x_2 = 0$, $x_2 = 1$, along with $x_1 = 0$) over the joint density $f_{X_1X_2}(x_1,x_2)$. I doubt you'll find this easier, but if you'd like to see it I can make another answer post.
– Lee David Chung Lin
Dec 4 at 11:06
1
Meanwhile, originally you posted the question with a snapshot. Which textbook is it? It's rather unlikely that the method of variable transformation (1-dim and 2-dim) is not covered, even if it's only a half-decent material.
– Lee David Chung Lin
Dec 4 at 11:38
Thank you very much for your response! Is there another way to solve this question? I don't think i understand the Jacobian method.
– OvermanZarathustra
Dec 3 at 18:23
Thank you very much for your response! Is there another way to solve this question? I don't think i understand the Jacobian method.
– OvermanZarathustra
Dec 3 at 18:23
1
1
It is a well-known basic fact that Beta distribution can be viewed as $frac{U}{U+W}$ where $U$ and $W$ are independent and follow Gamma distributions (of the same rate/scale parameter). See this or the 10th item of this. The question is just a symbolic (verbal) argument of the inverse of this definition. However, this way of quoting "known results" is hardly what the question seems to be aiming for.
– Lee David Chung Lin
Dec 4 at 11:01
It is a well-known basic fact that Beta distribution can be viewed as $frac{U}{U+W}$ where $U$ and $W$ are independent and follow Gamma distributions (of the same rate/scale parameter). See this or the 10th item of this. The question is just a symbolic (verbal) argument of the inverse of this definition. However, this way of quoting "known results" is hardly what the question seems to be aiming for.
– Lee David Chung Lin
Dec 4 at 11:01
1
1
The question statement basically gives you the joint $f_{X1X2}(x_1,x_2)$ and the intention is most likely asking you to do some calculus one way or the other. For example, you can do the CDF of $Y_1$ as $Pr{Y_1 < y_1 } = Pr{X_1 X_2 < y_1 }$, which is a 2-dim integration across the relevant region (bounded by the hyperbola $x_1 x_2 = y_1$ and $x_2 = 0$, $x_2 = 1$, along with $x_1 = 0$) over the joint density $f_{X_1X_2}(x_1,x_2)$. I doubt you'll find this easier, but if you'd like to see it I can make another answer post.
– Lee David Chung Lin
Dec 4 at 11:06
The question statement basically gives you the joint $f_{X1X2}(x_1,x_2)$ and the intention is most likely asking you to do some calculus one way or the other. For example, you can do the CDF of $Y_1$ as $Pr{Y_1 < y_1 } = Pr{X_1 X_2 < y_1 }$, which is a 2-dim integration across the relevant region (bounded by the hyperbola $x_1 x_2 = y_1$ and $x_2 = 0$, $x_2 = 1$, along with $x_1 = 0$) over the joint density $f_{X_1X_2}(x_1,x_2)$. I doubt you'll find this easier, but if you'd like to see it I can make another answer post.
– Lee David Chung Lin
Dec 4 at 11:06
1
1
Meanwhile, originally you posted the question with a snapshot. Which textbook is it? It's rather unlikely that the method of variable transformation (1-dim and 2-dim) is not covered, even if it's only a half-decent material.
– Lee David Chung Lin
Dec 4 at 11:38
Meanwhile, originally you posted the question with a snapshot. Which textbook is it? It's rather unlikely that the method of variable transformation (1-dim and 2-dim) is not covered, even if it's only a half-decent material.
– Lee David Chung Lin
Dec 4 at 11:38
add a comment |
Thanks for contributing an answer to Mathematics Stack Exchange!
- Please be sure to answer the question. Provide details and share your research!
But avoid …
- Asking for help, clarification, or responding to other answers.
- Making statements based on opinion; back them up with references or personal experience.
Use MathJax to format equations. MathJax reference.
To learn more, see our tips on writing great answers.
Some of your past answers have not been well-received, and you're in danger of being blocked from answering.
Please pay close attention to the following guidance:
- Please be sure to answer the question. Provide details and share your research!
But avoid …
- Asking for help, clarification, or responding to other answers.
- Making statements based on opinion; back them up with references or personal experience.
To learn more, see our tips on writing great answers.
Sign up or log in
StackExchange.ready(function () {
StackExchange.helpers.onClickDraftSave('#login-link');
});
Sign up using Google
Sign up using Facebook
Sign up using Email and Password
Post as a guest
Required, but never shown
StackExchange.ready(
function () {
StackExchange.openid.initPostLogin('.new-post-login', 'https%3a%2f%2fmath.stackexchange.com%2fquestions%2f3021216%2fmarginal-density-function-gamma-and-beta-distributions%23new-answer', 'question_page');
}
);
Post as a guest
Required, but never shown
Sign up or log in
StackExchange.ready(function () {
StackExchange.helpers.onClickDraftSave('#login-link');
});
Sign up using Google
Sign up using Facebook
Sign up using Email and Password
Post as a guest
Required, but never shown
Sign up or log in
StackExchange.ready(function () {
StackExchange.helpers.onClickDraftSave('#login-link');
});
Sign up using Google
Sign up using Facebook
Sign up using Email and Password
Post as a guest
Required, but never shown
Sign up or log in
StackExchange.ready(function () {
StackExchange.helpers.onClickDraftSave('#login-link');
});
Sign up using Google
Sign up using Facebook
Sign up using Email and Password
Sign up using Google
Sign up using Facebook
Sign up using Email and Password
Post as a guest
Required, but never shown
Required, but never shown
Required, but never shown
Required, but never shown
Required, but never shown
Required, but never shown
Required, but never shown
Required, but never shown
Required, but never shown
2u47W,knVGPtumQP3SIayFvpy RL6d6 DCjs1z20IX d9Vk
3
Perhaps you meant how to find the joint density of $(Y_1,Y_2)$. Do you know change of variables?
– StubbornAtom
Dec 1 at 10:55
@StubbornAtom thank you for your response. By change of variables you mean for integration?
– OvermanZarathustra
Dec 4 at 0:00