Tensorflow pre-trained embedding matrix part of graph
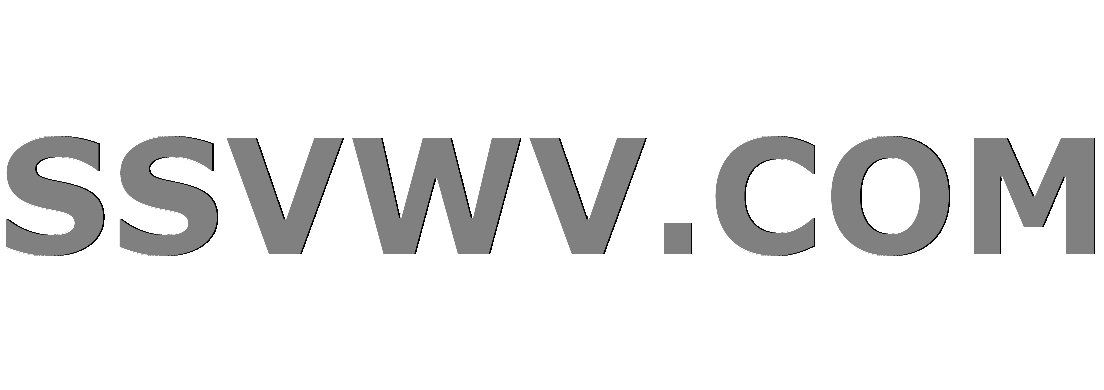
Multi tool use
I am currently trying to use my own pre-trained word embeddings in my tensorflow model_fn, but after a single epoch, the model building fails due to the following error;
ValueError: Fetch argument <tf.Variable 'embedding/embeddings:0' shape=(86565, 300) dtype=float32_ref> cannot be interpreted a
s a Tensor. (Tensor Tensor("embedding/embeddings:0", shape=(86565, 300), dtype=float32_ref) is not an element of this graph.)
How do I modify my code in order to maintain the embeddings in the graph over several epochs? Should I be initializing them in a different way? Or outside of the model function?
def word_embeddings_matrix():
..... load my embeddings .....
return embedding_matrix
embedding_matrix = word_embeddings_matrix()
def model_fn(features, labels, mode, params):
vocab_table = lookup.index_table_from_file(vocabulary_file='dataset/vocab.csv', num_oov_buckets=1, default_value=-1)
text = features[commons.FEATURE_COL]
words = tf.string_split(text)
dense_words = tf.sparse_tensor_to_dense(words, default_value=commons.PAD_WORD)
word_ids = vocab_table.lookup(dense_words)
padding = tf.constant([[0, 0], [0, commons.MAX_DOCUMENT_LENGTH]])
# Pad all the word_ids entries to the maximum document length
word_ids_padded = tf.pad(word_ids, padding)
word_id_vector = tf.slice(word_ids_padded, [0, 0], [-1, commons.MAX_DOCUMENT_LENGTH])
embedding_matrix = word_embeddings_matrix()
if mode == tf.estimator.ModeKeys.TRAIN:
tf.keras.backend.set_learning_phase(True)
else:
tf.keras.backend.set_learning_phase(False)
# embedded_sequences = tf.keras.layers.Embedding(params.N_WORDS,
# 10, input_length=commons.MAX_DOCUMENT_LENGTH)(word_id_vector)
embedded_sequences = tf.keras.layers.Embedding(params.N_WORDS,
300,
weights=[embedding_matrix],
input_length=commons.MAX_DOCUMENT_LENGTH,
trainable=True)(word_id_vector)
conv = tf.keras.layers.Conv1D(filters=64, kernel_size=2, activation='relu')(embedded_sequences)
pool = tf.keras.layers.GlobalAveragePooling1D()(conv)
drop = tf.keras.layers.Dropout(0.5)(pool)
logits = tf.keras.layers.Dense(commons.TARGET_SIZE, activation=None)(drop)
predictions = tf.nn.softmax(logits)
prediction_indices = tf.argmax(predictions, axis=1)
python tensorflow
add a comment |
I am currently trying to use my own pre-trained word embeddings in my tensorflow model_fn, but after a single epoch, the model building fails due to the following error;
ValueError: Fetch argument <tf.Variable 'embedding/embeddings:0' shape=(86565, 300) dtype=float32_ref> cannot be interpreted a
s a Tensor. (Tensor Tensor("embedding/embeddings:0", shape=(86565, 300), dtype=float32_ref) is not an element of this graph.)
How do I modify my code in order to maintain the embeddings in the graph over several epochs? Should I be initializing them in a different way? Or outside of the model function?
def word_embeddings_matrix():
..... load my embeddings .....
return embedding_matrix
embedding_matrix = word_embeddings_matrix()
def model_fn(features, labels, mode, params):
vocab_table = lookup.index_table_from_file(vocabulary_file='dataset/vocab.csv', num_oov_buckets=1, default_value=-1)
text = features[commons.FEATURE_COL]
words = tf.string_split(text)
dense_words = tf.sparse_tensor_to_dense(words, default_value=commons.PAD_WORD)
word_ids = vocab_table.lookup(dense_words)
padding = tf.constant([[0, 0], [0, commons.MAX_DOCUMENT_LENGTH]])
# Pad all the word_ids entries to the maximum document length
word_ids_padded = tf.pad(word_ids, padding)
word_id_vector = tf.slice(word_ids_padded, [0, 0], [-1, commons.MAX_DOCUMENT_LENGTH])
embedding_matrix = word_embeddings_matrix()
if mode == tf.estimator.ModeKeys.TRAIN:
tf.keras.backend.set_learning_phase(True)
else:
tf.keras.backend.set_learning_phase(False)
# embedded_sequences = tf.keras.layers.Embedding(params.N_WORDS,
# 10, input_length=commons.MAX_DOCUMENT_LENGTH)(word_id_vector)
embedded_sequences = tf.keras.layers.Embedding(params.N_WORDS,
300,
weights=[embedding_matrix],
input_length=commons.MAX_DOCUMENT_LENGTH,
trainable=True)(word_id_vector)
conv = tf.keras.layers.Conv1D(filters=64, kernel_size=2, activation='relu')(embedded_sequences)
pool = tf.keras.layers.GlobalAveragePooling1D()(conv)
drop = tf.keras.layers.Dropout(0.5)(pool)
logits = tf.keras.layers.Dense(commons.TARGET_SIZE, activation=None)(drop)
predictions = tf.nn.softmax(logits)
prediction_indices = tf.argmax(predictions, axis=1)
python tensorflow
add a comment |
I am currently trying to use my own pre-trained word embeddings in my tensorflow model_fn, but after a single epoch, the model building fails due to the following error;
ValueError: Fetch argument <tf.Variable 'embedding/embeddings:0' shape=(86565, 300) dtype=float32_ref> cannot be interpreted a
s a Tensor. (Tensor Tensor("embedding/embeddings:0", shape=(86565, 300), dtype=float32_ref) is not an element of this graph.)
How do I modify my code in order to maintain the embeddings in the graph over several epochs? Should I be initializing them in a different way? Or outside of the model function?
def word_embeddings_matrix():
..... load my embeddings .....
return embedding_matrix
embedding_matrix = word_embeddings_matrix()
def model_fn(features, labels, mode, params):
vocab_table = lookup.index_table_from_file(vocabulary_file='dataset/vocab.csv', num_oov_buckets=1, default_value=-1)
text = features[commons.FEATURE_COL]
words = tf.string_split(text)
dense_words = tf.sparse_tensor_to_dense(words, default_value=commons.PAD_WORD)
word_ids = vocab_table.lookup(dense_words)
padding = tf.constant([[0, 0], [0, commons.MAX_DOCUMENT_LENGTH]])
# Pad all the word_ids entries to the maximum document length
word_ids_padded = tf.pad(word_ids, padding)
word_id_vector = tf.slice(word_ids_padded, [0, 0], [-1, commons.MAX_DOCUMENT_LENGTH])
embedding_matrix = word_embeddings_matrix()
if mode == tf.estimator.ModeKeys.TRAIN:
tf.keras.backend.set_learning_phase(True)
else:
tf.keras.backend.set_learning_phase(False)
# embedded_sequences = tf.keras.layers.Embedding(params.N_WORDS,
# 10, input_length=commons.MAX_DOCUMENT_LENGTH)(word_id_vector)
embedded_sequences = tf.keras.layers.Embedding(params.N_WORDS,
300,
weights=[embedding_matrix],
input_length=commons.MAX_DOCUMENT_LENGTH,
trainable=True)(word_id_vector)
conv = tf.keras.layers.Conv1D(filters=64, kernel_size=2, activation='relu')(embedded_sequences)
pool = tf.keras.layers.GlobalAveragePooling1D()(conv)
drop = tf.keras.layers.Dropout(0.5)(pool)
logits = tf.keras.layers.Dense(commons.TARGET_SIZE, activation=None)(drop)
predictions = tf.nn.softmax(logits)
prediction_indices = tf.argmax(predictions, axis=1)
python tensorflow
I am currently trying to use my own pre-trained word embeddings in my tensorflow model_fn, but after a single epoch, the model building fails due to the following error;
ValueError: Fetch argument <tf.Variable 'embedding/embeddings:0' shape=(86565, 300) dtype=float32_ref> cannot be interpreted a
s a Tensor. (Tensor Tensor("embedding/embeddings:0", shape=(86565, 300), dtype=float32_ref) is not an element of this graph.)
How do I modify my code in order to maintain the embeddings in the graph over several epochs? Should I be initializing them in a different way? Or outside of the model function?
def word_embeddings_matrix():
..... load my embeddings .....
return embedding_matrix
embedding_matrix = word_embeddings_matrix()
def model_fn(features, labels, mode, params):
vocab_table = lookup.index_table_from_file(vocabulary_file='dataset/vocab.csv', num_oov_buckets=1, default_value=-1)
text = features[commons.FEATURE_COL]
words = tf.string_split(text)
dense_words = tf.sparse_tensor_to_dense(words, default_value=commons.PAD_WORD)
word_ids = vocab_table.lookup(dense_words)
padding = tf.constant([[0, 0], [0, commons.MAX_DOCUMENT_LENGTH]])
# Pad all the word_ids entries to the maximum document length
word_ids_padded = tf.pad(word_ids, padding)
word_id_vector = tf.slice(word_ids_padded, [0, 0], [-1, commons.MAX_DOCUMENT_LENGTH])
embedding_matrix = word_embeddings_matrix()
if mode == tf.estimator.ModeKeys.TRAIN:
tf.keras.backend.set_learning_phase(True)
else:
tf.keras.backend.set_learning_phase(False)
# embedded_sequences = tf.keras.layers.Embedding(params.N_WORDS,
# 10, input_length=commons.MAX_DOCUMENT_LENGTH)(word_id_vector)
embedded_sequences = tf.keras.layers.Embedding(params.N_WORDS,
300,
weights=[embedding_matrix],
input_length=commons.MAX_DOCUMENT_LENGTH,
trainable=True)(word_id_vector)
conv = tf.keras.layers.Conv1D(filters=64, kernel_size=2, activation='relu')(embedded_sequences)
pool = tf.keras.layers.GlobalAveragePooling1D()(conv)
drop = tf.keras.layers.Dropout(0.5)(pool)
logits = tf.keras.layers.Dense(commons.TARGET_SIZE, activation=None)(drop)
predictions = tf.nn.softmax(logits)
prediction_indices = tf.argmax(predictions, axis=1)
python tensorflow
python tensorflow
asked Nov 23 '18 at 16:00
chattrat423chattrat423
2631316
2631316
add a comment |
add a comment |
0
active
oldest
votes
Your Answer
StackExchange.ifUsing("editor", function () {
StackExchange.using("externalEditor", function () {
StackExchange.using("snippets", function () {
StackExchange.snippets.init();
});
});
}, "code-snippets");
StackExchange.ready(function() {
var channelOptions = {
tags: "".split(" "),
id: "1"
};
initTagRenderer("".split(" "), "".split(" "), channelOptions);
StackExchange.using("externalEditor", function() {
// Have to fire editor after snippets, if snippets enabled
if (StackExchange.settings.snippets.snippetsEnabled) {
StackExchange.using("snippets", function() {
createEditor();
});
}
else {
createEditor();
}
});
function createEditor() {
StackExchange.prepareEditor({
heartbeatType: 'answer',
autoActivateHeartbeat: false,
convertImagesToLinks: true,
noModals: true,
showLowRepImageUploadWarning: true,
reputationToPostImages: 10,
bindNavPrevention: true,
postfix: "",
imageUploader: {
brandingHtml: "Powered by u003ca class="icon-imgur-white" href="https://imgur.com/"u003eu003c/au003e",
contentPolicyHtml: "User contributions licensed under u003ca href="https://creativecommons.org/licenses/by-sa/3.0/"u003ecc by-sa 3.0 with attribution requiredu003c/au003e u003ca href="https://stackoverflow.com/legal/content-policy"u003e(content policy)u003c/au003e",
allowUrls: true
},
onDemand: true,
discardSelector: ".discard-answer"
,immediatelyShowMarkdownHelp:true
});
}
});
Sign up or log in
StackExchange.ready(function () {
StackExchange.helpers.onClickDraftSave('#login-link');
});
Sign up using Google
Sign up using Facebook
Sign up using Email and Password
Post as a guest
Required, but never shown
StackExchange.ready(
function () {
StackExchange.openid.initPostLogin('.new-post-login', 'https%3a%2f%2fstackoverflow.com%2fquestions%2f53449742%2ftensorflow-pre-trained-embedding-matrix-part-of-graph%23new-answer', 'question_page');
}
);
Post as a guest
Required, but never shown
0
active
oldest
votes
0
active
oldest
votes
active
oldest
votes
active
oldest
votes
Thanks for contributing an answer to Stack Overflow!
- Please be sure to answer the question. Provide details and share your research!
But avoid …
- Asking for help, clarification, or responding to other answers.
- Making statements based on opinion; back them up with references or personal experience.
To learn more, see our tips on writing great answers.
Sign up or log in
StackExchange.ready(function () {
StackExchange.helpers.onClickDraftSave('#login-link');
});
Sign up using Google
Sign up using Facebook
Sign up using Email and Password
Post as a guest
Required, but never shown
StackExchange.ready(
function () {
StackExchange.openid.initPostLogin('.new-post-login', 'https%3a%2f%2fstackoverflow.com%2fquestions%2f53449742%2ftensorflow-pre-trained-embedding-matrix-part-of-graph%23new-answer', 'question_page');
}
);
Post as a guest
Required, but never shown
Sign up or log in
StackExchange.ready(function () {
StackExchange.helpers.onClickDraftSave('#login-link');
});
Sign up using Google
Sign up using Facebook
Sign up using Email and Password
Post as a guest
Required, but never shown
Sign up or log in
StackExchange.ready(function () {
StackExchange.helpers.onClickDraftSave('#login-link');
});
Sign up using Google
Sign up using Facebook
Sign up using Email and Password
Post as a guest
Required, but never shown
Sign up or log in
StackExchange.ready(function () {
StackExchange.helpers.onClickDraftSave('#login-link');
});
Sign up using Google
Sign up using Facebook
Sign up using Email and Password
Sign up using Google
Sign up using Facebook
Sign up using Email and Password
Post as a guest
Required, but never shown
Required, but never shown
Required, but never shown
Required, but never shown
Required, but never shown
Required, but never shown
Required, but never shown
Required, but never shown
Required, but never shown
B DHYlV3H3CiwoMy1yrP1b GTeBKfceNY3F,iY b9YZn3N,mZrwVXSQntY8hOFtr0cT,IrRmqpRw VZX2T,AHvdy ky