On the limit of a continuous combination of sequences of random variables converging in distribution
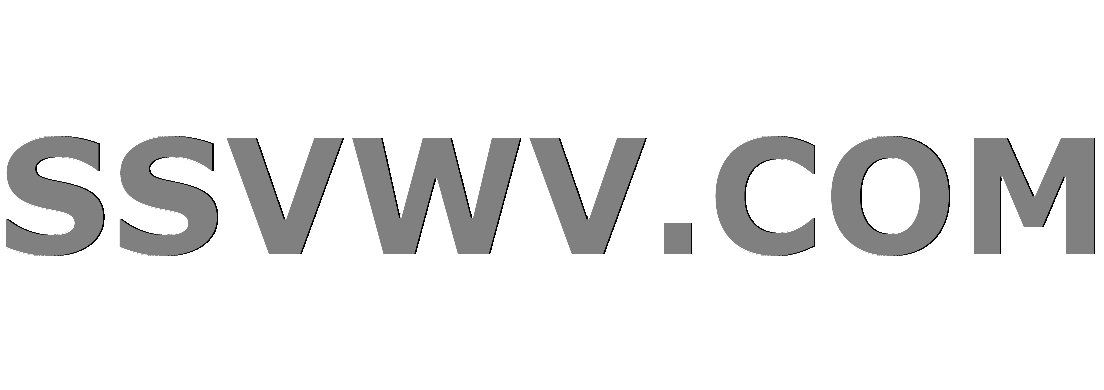
Multi tool use
up vote
1
down vote
favorite
Let ${X_n}, {Y_n}$ be sequences of real valued random variables converging in distribution to $X$ and $Y$ respectively. Let $f: mathbb R^2 to mathbb R$ be a continuous function such that ${f(X_n,Y_n)}$ converges in distribution to some random variable $Z$.
Then is it true that $Z$ is identically distributed as $f(X,Y)$ ?
If this is not true in general, is it at least true for the function $f(x,y)=x+y$ ?
probability-theory measure-theory probability-distributions random-variables
add a comment |
up vote
1
down vote
favorite
Let ${X_n}, {Y_n}$ be sequences of real valued random variables converging in distribution to $X$ and $Y$ respectively. Let $f: mathbb R^2 to mathbb R$ be a continuous function such that ${f(X_n,Y_n)}$ converges in distribution to some random variable $Z$.
Then is it true that $Z$ is identically distributed as $f(X,Y)$ ?
If this is not true in general, is it at least true for the function $f(x,y)=x+y$ ?
probability-theory measure-theory probability-distributions random-variables
add a comment |
up vote
1
down vote
favorite
up vote
1
down vote
favorite
Let ${X_n}, {Y_n}$ be sequences of real valued random variables converging in distribution to $X$ and $Y$ respectively. Let $f: mathbb R^2 to mathbb R$ be a continuous function such that ${f(X_n,Y_n)}$ converges in distribution to some random variable $Z$.
Then is it true that $Z$ is identically distributed as $f(X,Y)$ ?
If this is not true in general, is it at least true for the function $f(x,y)=x+y$ ?
probability-theory measure-theory probability-distributions random-variables
Let ${X_n}, {Y_n}$ be sequences of real valued random variables converging in distribution to $X$ and $Y$ respectively. Let $f: mathbb R^2 to mathbb R$ be a continuous function such that ${f(X_n,Y_n)}$ converges in distribution to some random variable $Z$.
Then is it true that $Z$ is identically distributed as $f(X,Y)$ ?
If this is not true in general, is it at least true for the function $f(x,y)=x+y$ ?
probability-theory measure-theory probability-distributions random-variables
probability-theory measure-theory probability-distributions random-variables
asked yesterday
user521337
491113
491113
add a comment |
add a comment |
2 Answers
2
active
oldest
votes
up vote
1
down vote
accepted
Not without independence assumptions (or convergence of joint distributions). Take ${X_n}$ i.i.d. with standard normal distribution, $Y_n=-X_n$ for all $n$ and $f(x,y)=x+y$. Then ${X_n} to X_1$ and ${Y_n} to X_1$ in distribution but $X_n+Y_n to 0$ in distribution.
add a comment |
up vote
0
down vote
For your question to make sense, you need to mention that the vectors $(X,Y)$ and $(X_n, Y_n)$ exist (i.e. $X_n$ and $Y_n$ must live in the same probability space).
If you have the stronger assumption that $(X_n, Y_n)$ converges in distribution to the vector $(X,Y)$, then $f(X_n, Y_n)$ converges in distribution to $f(X,Y)$ by the continuous mapping theorem.
Without this stronger assumption, the claim does not hold, even for $f(x,y) = x + y$, as demonstrated in one of the answers in this question.
add a comment |
2 Answers
2
active
oldest
votes
2 Answers
2
active
oldest
votes
active
oldest
votes
active
oldest
votes
up vote
1
down vote
accepted
Not without independence assumptions (or convergence of joint distributions). Take ${X_n}$ i.i.d. with standard normal distribution, $Y_n=-X_n$ for all $n$ and $f(x,y)=x+y$. Then ${X_n} to X_1$ and ${Y_n} to X_1$ in distribution but $X_n+Y_n to 0$ in distribution.
add a comment |
up vote
1
down vote
accepted
Not without independence assumptions (or convergence of joint distributions). Take ${X_n}$ i.i.d. with standard normal distribution, $Y_n=-X_n$ for all $n$ and $f(x,y)=x+y$. Then ${X_n} to X_1$ and ${Y_n} to X_1$ in distribution but $X_n+Y_n to 0$ in distribution.
add a comment |
up vote
1
down vote
accepted
up vote
1
down vote
accepted
Not without independence assumptions (or convergence of joint distributions). Take ${X_n}$ i.i.d. with standard normal distribution, $Y_n=-X_n$ for all $n$ and $f(x,y)=x+y$. Then ${X_n} to X_1$ and ${Y_n} to X_1$ in distribution but $X_n+Y_n to 0$ in distribution.
Not without independence assumptions (or convergence of joint distributions). Take ${X_n}$ i.i.d. with standard normal distribution, $Y_n=-X_n$ for all $n$ and $f(x,y)=x+y$. Then ${X_n} to X_1$ and ${Y_n} to X_1$ in distribution but $X_n+Y_n to 0$ in distribution.
answered yesterday


Kavi Rama Murthy
40.7k31751
40.7k31751
add a comment |
add a comment |
up vote
0
down vote
For your question to make sense, you need to mention that the vectors $(X,Y)$ and $(X_n, Y_n)$ exist (i.e. $X_n$ and $Y_n$ must live in the same probability space).
If you have the stronger assumption that $(X_n, Y_n)$ converges in distribution to the vector $(X,Y)$, then $f(X_n, Y_n)$ converges in distribution to $f(X,Y)$ by the continuous mapping theorem.
Without this stronger assumption, the claim does not hold, even for $f(x,y) = x + y$, as demonstrated in one of the answers in this question.
add a comment |
up vote
0
down vote
For your question to make sense, you need to mention that the vectors $(X,Y)$ and $(X_n, Y_n)$ exist (i.e. $X_n$ and $Y_n$ must live in the same probability space).
If you have the stronger assumption that $(X_n, Y_n)$ converges in distribution to the vector $(X,Y)$, then $f(X_n, Y_n)$ converges in distribution to $f(X,Y)$ by the continuous mapping theorem.
Without this stronger assumption, the claim does not hold, even for $f(x,y) = x + y$, as demonstrated in one of the answers in this question.
add a comment |
up vote
0
down vote
up vote
0
down vote
For your question to make sense, you need to mention that the vectors $(X,Y)$ and $(X_n, Y_n)$ exist (i.e. $X_n$ and $Y_n$ must live in the same probability space).
If you have the stronger assumption that $(X_n, Y_n)$ converges in distribution to the vector $(X,Y)$, then $f(X_n, Y_n)$ converges in distribution to $f(X,Y)$ by the continuous mapping theorem.
Without this stronger assumption, the claim does not hold, even for $f(x,y) = x + y$, as demonstrated in one of the answers in this question.
For your question to make sense, you need to mention that the vectors $(X,Y)$ and $(X_n, Y_n)$ exist (i.e. $X_n$ and $Y_n$ must live in the same probability space).
If you have the stronger assumption that $(X_n, Y_n)$ converges in distribution to the vector $(X,Y)$, then $f(X_n, Y_n)$ converges in distribution to $f(X,Y)$ by the continuous mapping theorem.
Without this stronger assumption, the claim does not hold, even for $f(x,y) = x + y$, as demonstrated in one of the answers in this question.
answered yesterday
angryavian
36.9k13178
36.9k13178
add a comment |
add a comment |
Sign up or log in
StackExchange.ready(function () {
StackExchange.helpers.onClickDraftSave('#login-link');
});
Sign up using Google
Sign up using Facebook
Sign up using Email and Password
Post as a guest
Required, but never shown
StackExchange.ready(
function () {
StackExchange.openid.initPostLogin('.new-post-login', 'https%3a%2f%2fmath.stackexchange.com%2fquestions%2f3007033%2fon-the-limit-of-a-continuous-combination-of-sequences-of-random-variables-conver%23new-answer', 'question_page');
}
);
Post as a guest
Required, but never shown
Sign up or log in
StackExchange.ready(function () {
StackExchange.helpers.onClickDraftSave('#login-link');
});
Sign up using Google
Sign up using Facebook
Sign up using Email and Password
Post as a guest
Required, but never shown
Sign up or log in
StackExchange.ready(function () {
StackExchange.helpers.onClickDraftSave('#login-link');
});
Sign up using Google
Sign up using Facebook
Sign up using Email and Password
Post as a guest
Required, but never shown
Sign up or log in
StackExchange.ready(function () {
StackExchange.helpers.onClickDraftSave('#login-link');
});
Sign up using Google
Sign up using Facebook
Sign up using Email and Password
Sign up using Google
Sign up using Facebook
Sign up using Email and Password
Post as a guest
Required, but never shown
Required, but never shown
Required, but never shown
Required, but never shown
Required, but never shown
Required, but never shown
Required, but never shown
Required, but never shown
Required, but never shown
84qsNyCfjBDAQIP9L eONjgJsHVwJX zMC,k0T04PvLdE7QkU,rnlZPLmZ,7NzZsjI4lyMUfwYl,l 1dRG8MwQ2 gz pGOnSlOwHcy