1D signal data processing
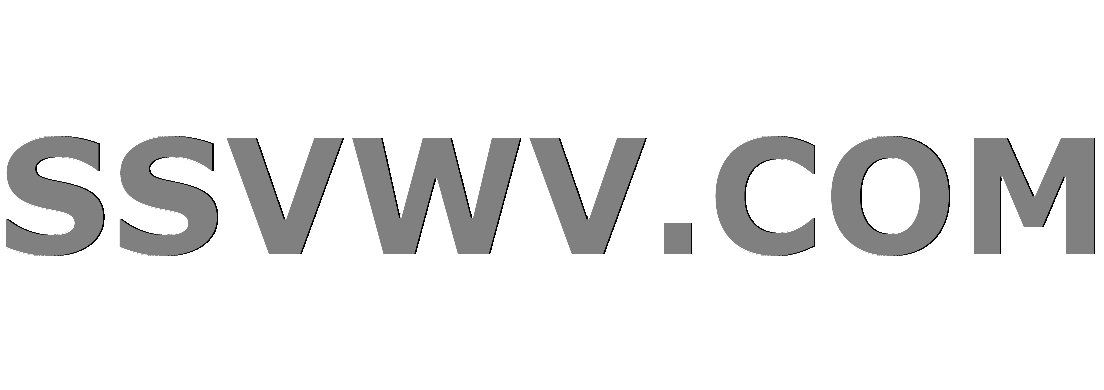
Multi tool use
up vote
1
down vote
favorite
I have 1D signal data which I am provided, its basically synthetic data, not realistic.
I have 1000 CSV files for the same which have the following variables.
1=depth(index), 2=signal value(1D signal), 3=event id, 4=event id as a class (target variable), 5=event and class (not useful)
0 , 3 , 0 , 0 , 0
1 , 5 , 0 , 0 , 0
2 , 2 , 0 , 0 , 0
3 , 3 , 1 , 1 , 1000
4 , 6 , 0 , 1 , 1
5 , 1 , 0 , 1 , 1
6 , 2 , 2 , 2 , 2000
7 , 6 , 0 , 2 , 2
8 , 2 , 0 , 2 , 2
9 , 6 , 0 , 2 , 2
I want to train multiple classification models using keras, pytorch, seq2seq.
I haveing various idea in my mind how to create the data so it looks better and feed them in my TCN/CNN networks.
feeding as a 1D array as feature and 1D array as label in a classification model does not make a good sense.
I need some ideas, if someone has done it before.
Thanks in Advance.
python pandas numpy signal-processing
add a comment |
up vote
1
down vote
favorite
I have 1D signal data which I am provided, its basically synthetic data, not realistic.
I have 1000 CSV files for the same which have the following variables.
1=depth(index), 2=signal value(1D signal), 3=event id, 4=event id as a class (target variable), 5=event and class (not useful)
0 , 3 , 0 , 0 , 0
1 , 5 , 0 , 0 , 0
2 , 2 , 0 , 0 , 0
3 , 3 , 1 , 1 , 1000
4 , 6 , 0 , 1 , 1
5 , 1 , 0 , 1 , 1
6 , 2 , 2 , 2 , 2000
7 , 6 , 0 , 2 , 2
8 , 2 , 0 , 2 , 2
9 , 6 , 0 , 2 , 2
I want to train multiple classification models using keras, pytorch, seq2seq.
I haveing various idea in my mind how to create the data so it looks better and feed them in my TCN/CNN networks.
feeding as a 1D array as feature and 1D array as label in a classification model does not make a good sense.
I need some ideas, if someone has done it before.
Thanks in Advance.
python pandas numpy signal-processing
add a comment |
up vote
1
down vote
favorite
up vote
1
down vote
favorite
I have 1D signal data which I am provided, its basically synthetic data, not realistic.
I have 1000 CSV files for the same which have the following variables.
1=depth(index), 2=signal value(1D signal), 3=event id, 4=event id as a class (target variable), 5=event and class (not useful)
0 , 3 , 0 , 0 , 0
1 , 5 , 0 , 0 , 0
2 , 2 , 0 , 0 , 0
3 , 3 , 1 , 1 , 1000
4 , 6 , 0 , 1 , 1
5 , 1 , 0 , 1 , 1
6 , 2 , 2 , 2 , 2000
7 , 6 , 0 , 2 , 2
8 , 2 , 0 , 2 , 2
9 , 6 , 0 , 2 , 2
I want to train multiple classification models using keras, pytorch, seq2seq.
I haveing various idea in my mind how to create the data so it looks better and feed them in my TCN/CNN networks.
feeding as a 1D array as feature and 1D array as label in a classification model does not make a good sense.
I need some ideas, if someone has done it before.
Thanks in Advance.
python pandas numpy signal-processing
I have 1D signal data which I am provided, its basically synthetic data, not realistic.
I have 1000 CSV files for the same which have the following variables.
1=depth(index), 2=signal value(1D signal), 3=event id, 4=event id as a class (target variable), 5=event and class (not useful)
0 , 3 , 0 , 0 , 0
1 , 5 , 0 , 0 , 0
2 , 2 , 0 , 0 , 0
3 , 3 , 1 , 1 , 1000
4 , 6 , 0 , 1 , 1
5 , 1 , 0 , 1 , 1
6 , 2 , 2 , 2 , 2000
7 , 6 , 0 , 2 , 2
8 , 2 , 0 , 2 , 2
9 , 6 , 0 , 2 , 2
I want to train multiple classification models using keras, pytorch, seq2seq.
I haveing various idea in my mind how to create the data so it looks better and feed them in my TCN/CNN networks.
feeding as a 1D array as feature and 1D array as label in a classification model does not make a good sense.
I need some ideas, if someone has done it before.
Thanks in Advance.
python pandas numpy signal-processing
python pandas numpy signal-processing
asked Nov 21 at 17:54


Shivam_hbti
376
376
add a comment |
add a comment |
active
oldest
votes
active
oldest
votes
active
oldest
votes
active
oldest
votes
active
oldest
votes
Thanks for contributing an answer to Stack Overflow!
- Please be sure to answer the question. Provide details and share your research!
But avoid …
- Asking for help, clarification, or responding to other answers.
- Making statements based on opinion; back them up with references or personal experience.
To learn more, see our tips on writing great answers.
Some of your past answers have not been well-received, and you're in danger of being blocked from answering.
Please pay close attention to the following guidance:
- Please be sure to answer the question. Provide details and share your research!
But avoid …
- Asking for help, clarification, or responding to other answers.
- Making statements based on opinion; back them up with references or personal experience.
To learn more, see our tips on writing great answers.
Sign up or log in
StackExchange.ready(function () {
StackExchange.helpers.onClickDraftSave('#login-link');
});
Sign up using Google
Sign up using Facebook
Sign up using Email and Password
Post as a guest
Required, but never shown
StackExchange.ready(
function () {
StackExchange.openid.initPostLogin('.new-post-login', 'https%3a%2f%2fstackoverflow.com%2fquestions%2f53417959%2f1d-signal-data-processing%23new-answer', 'question_page');
}
);
Post as a guest
Required, but never shown
Sign up or log in
StackExchange.ready(function () {
StackExchange.helpers.onClickDraftSave('#login-link');
});
Sign up using Google
Sign up using Facebook
Sign up using Email and Password
Post as a guest
Required, but never shown
Sign up or log in
StackExchange.ready(function () {
StackExchange.helpers.onClickDraftSave('#login-link');
});
Sign up using Google
Sign up using Facebook
Sign up using Email and Password
Post as a guest
Required, but never shown
Sign up or log in
StackExchange.ready(function () {
StackExchange.helpers.onClickDraftSave('#login-link');
});
Sign up using Google
Sign up using Facebook
Sign up using Email and Password
Sign up using Google
Sign up using Facebook
Sign up using Email and Password
Post as a guest
Required, but never shown
Required, but never shown
Required, but never shown
Required, but never shown
Required, but never shown
Required, but never shown
Required, but never shown
Required, but never shown
Required, but never shown
XwZQ 2Co hunHvEva,J8K,V0r50B