understanding seed of a ByteTensor in PyTorch
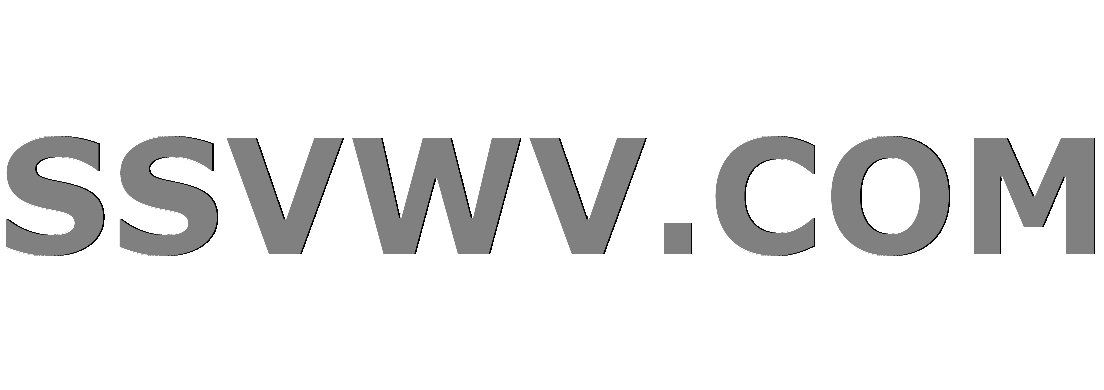
Multi tool use
I understand that a seed is a number used to initialize pseudo-random number generator. in pytorch, torch.get_rng_state
documentation states as follows "Returns the random number generator state as a torch.ByteTensor.". and when i print it i get a 1-d tensor of size 5048 whose values are as shown below
tensor([ 80, 78, 248, ..., 0, 0, 0], dtype=torch.uint8)
why does a seed have 5048 values and how is this different from usual seed which we can get using torch.initial_seed
random pytorch random-seed
add a comment |
I understand that a seed is a number used to initialize pseudo-random number generator. in pytorch, torch.get_rng_state
documentation states as follows "Returns the random number generator state as a torch.ByteTensor.". and when i print it i get a 1-d tensor of size 5048 whose values are as shown below
tensor([ 80, 78, 248, ..., 0, 0, 0], dtype=torch.uint8)
why does a seed have 5048 values and how is this different from usual seed which we can get using torch.initial_seed
random pytorch random-seed
add a comment |
I understand that a seed is a number used to initialize pseudo-random number generator. in pytorch, torch.get_rng_state
documentation states as follows "Returns the random number generator state as a torch.ByteTensor.". and when i print it i get a 1-d tensor of size 5048 whose values are as shown below
tensor([ 80, 78, 248, ..., 0, 0, 0], dtype=torch.uint8)
why does a seed have 5048 values and how is this different from usual seed which we can get using torch.initial_seed
random pytorch random-seed
I understand that a seed is a number used to initialize pseudo-random number generator. in pytorch, torch.get_rng_state
documentation states as follows "Returns the random number generator state as a torch.ByteTensor.". and when i print it i get a 1-d tensor of size 5048 whose values are as shown below
tensor([ 80, 78, 248, ..., 0, 0, 0], dtype=torch.uint8)
why does a seed have 5048 values and how is this different from usual seed which we can get using torch.initial_seed
random pytorch random-seed
random pytorch random-seed
asked Nov 23 '18 at 19:27


InAFlashInAFlash
2,4722928
2,4722928
add a comment |
add a comment |
1 Answer
1
active
oldest
votes
It sounds like you're thinking of the seed and the state as equivalent. For older pseudo-random number generators (PRNGs) that was true, but with more modern PRNGs tend to work as described here. (The answer in the link was written with respect to Mersenne Twister, but the concepts apply equally to other generators.)
Why is it a good idea to not have a 32- or 64-bit state space and report the state as the generator's output? Because if you do that, as soon as you see any value repeat the entire sequence will repeat. PRNGs were designed to be "full cycle," i.e., to iterate through the maximum number of values possible before repeating. This paper showed that the birthday problem could quickly (O(sqrt(cycle-length)) identify such PRNGs as non-random. This meant, for instance, that with 32-bit integers you shouldn't use more than ~50000 values before a statistician could call you out with a better than 99% level of confidence. The solution, used by many modern PRNGs, is to have a larger state space and collapse it down to output a 32- or 64-bit result. Since multiple states can produce the same output, duplicates will occur in the output stream without the entire stream being replicated. It looks like that's what PyTorch is doing.
Given the larger state space, why allow seeding with a single integer? Convenience. For instance, Mersenne Twister has a 19,937 bit state space, but most people don't want to enter that much info to kick-start it. You can if you want to, but most people use the front-end which populates the full state space from a single integer input.
add a comment |
Your Answer
StackExchange.ifUsing("editor", function () {
StackExchange.using("externalEditor", function () {
StackExchange.using("snippets", function () {
StackExchange.snippets.init();
});
});
}, "code-snippets");
StackExchange.ready(function() {
var channelOptions = {
tags: "".split(" "),
id: "1"
};
initTagRenderer("".split(" "), "".split(" "), channelOptions);
StackExchange.using("externalEditor", function() {
// Have to fire editor after snippets, if snippets enabled
if (StackExchange.settings.snippets.snippetsEnabled) {
StackExchange.using("snippets", function() {
createEditor();
});
}
else {
createEditor();
}
});
function createEditor() {
StackExchange.prepareEditor({
heartbeatType: 'answer',
autoActivateHeartbeat: false,
convertImagesToLinks: true,
noModals: true,
showLowRepImageUploadWarning: true,
reputationToPostImages: 10,
bindNavPrevention: true,
postfix: "",
imageUploader: {
brandingHtml: "Powered by u003ca class="icon-imgur-white" href="https://imgur.com/"u003eu003c/au003e",
contentPolicyHtml: "User contributions licensed under u003ca href="https://creativecommons.org/licenses/by-sa/3.0/"u003ecc by-sa 3.0 with attribution requiredu003c/au003e u003ca href="https://stackoverflow.com/legal/content-policy"u003e(content policy)u003c/au003e",
allowUrls: true
},
onDemand: true,
discardSelector: ".discard-answer"
,immediatelyShowMarkdownHelp:true
});
}
});
Sign up or log in
StackExchange.ready(function () {
StackExchange.helpers.onClickDraftSave('#login-link');
});
Sign up using Google
Sign up using Facebook
Sign up using Email and Password
Post as a guest
Required, but never shown
StackExchange.ready(
function () {
StackExchange.openid.initPostLogin('.new-post-login', 'https%3a%2f%2fstackoverflow.com%2fquestions%2f53452049%2funderstanding-seed-of-a-bytetensor-in-pytorch%23new-answer', 'question_page');
}
);
Post as a guest
Required, but never shown
1 Answer
1
active
oldest
votes
1 Answer
1
active
oldest
votes
active
oldest
votes
active
oldest
votes
It sounds like you're thinking of the seed and the state as equivalent. For older pseudo-random number generators (PRNGs) that was true, but with more modern PRNGs tend to work as described here. (The answer in the link was written with respect to Mersenne Twister, but the concepts apply equally to other generators.)
Why is it a good idea to not have a 32- or 64-bit state space and report the state as the generator's output? Because if you do that, as soon as you see any value repeat the entire sequence will repeat. PRNGs were designed to be "full cycle," i.e., to iterate through the maximum number of values possible before repeating. This paper showed that the birthday problem could quickly (O(sqrt(cycle-length)) identify such PRNGs as non-random. This meant, for instance, that with 32-bit integers you shouldn't use more than ~50000 values before a statistician could call you out with a better than 99% level of confidence. The solution, used by many modern PRNGs, is to have a larger state space and collapse it down to output a 32- or 64-bit result. Since multiple states can produce the same output, duplicates will occur in the output stream without the entire stream being replicated. It looks like that's what PyTorch is doing.
Given the larger state space, why allow seeding with a single integer? Convenience. For instance, Mersenne Twister has a 19,937 bit state space, but most people don't want to enter that much info to kick-start it. You can if you want to, but most people use the front-end which populates the full state space from a single integer input.
add a comment |
It sounds like you're thinking of the seed and the state as equivalent. For older pseudo-random number generators (PRNGs) that was true, but with more modern PRNGs tend to work as described here. (The answer in the link was written with respect to Mersenne Twister, but the concepts apply equally to other generators.)
Why is it a good idea to not have a 32- or 64-bit state space and report the state as the generator's output? Because if you do that, as soon as you see any value repeat the entire sequence will repeat. PRNGs were designed to be "full cycle," i.e., to iterate through the maximum number of values possible before repeating. This paper showed that the birthday problem could quickly (O(sqrt(cycle-length)) identify such PRNGs as non-random. This meant, for instance, that with 32-bit integers you shouldn't use more than ~50000 values before a statistician could call you out with a better than 99% level of confidence. The solution, used by many modern PRNGs, is to have a larger state space and collapse it down to output a 32- or 64-bit result. Since multiple states can produce the same output, duplicates will occur in the output stream without the entire stream being replicated. It looks like that's what PyTorch is doing.
Given the larger state space, why allow seeding with a single integer? Convenience. For instance, Mersenne Twister has a 19,937 bit state space, but most people don't want to enter that much info to kick-start it. You can if you want to, but most people use the front-end which populates the full state space from a single integer input.
add a comment |
It sounds like you're thinking of the seed and the state as equivalent. For older pseudo-random number generators (PRNGs) that was true, but with more modern PRNGs tend to work as described here. (The answer in the link was written with respect to Mersenne Twister, but the concepts apply equally to other generators.)
Why is it a good idea to not have a 32- or 64-bit state space and report the state as the generator's output? Because if you do that, as soon as you see any value repeat the entire sequence will repeat. PRNGs were designed to be "full cycle," i.e., to iterate through the maximum number of values possible before repeating. This paper showed that the birthday problem could quickly (O(sqrt(cycle-length)) identify such PRNGs as non-random. This meant, for instance, that with 32-bit integers you shouldn't use more than ~50000 values before a statistician could call you out with a better than 99% level of confidence. The solution, used by many modern PRNGs, is to have a larger state space and collapse it down to output a 32- or 64-bit result. Since multiple states can produce the same output, duplicates will occur in the output stream without the entire stream being replicated. It looks like that's what PyTorch is doing.
Given the larger state space, why allow seeding with a single integer? Convenience. For instance, Mersenne Twister has a 19,937 bit state space, but most people don't want to enter that much info to kick-start it. You can if you want to, but most people use the front-end which populates the full state space from a single integer input.
It sounds like you're thinking of the seed and the state as equivalent. For older pseudo-random number generators (PRNGs) that was true, but with more modern PRNGs tend to work as described here. (The answer in the link was written with respect to Mersenne Twister, but the concepts apply equally to other generators.)
Why is it a good idea to not have a 32- or 64-bit state space and report the state as the generator's output? Because if you do that, as soon as you see any value repeat the entire sequence will repeat. PRNGs were designed to be "full cycle," i.e., to iterate through the maximum number of values possible before repeating. This paper showed that the birthday problem could quickly (O(sqrt(cycle-length)) identify such PRNGs as non-random. This meant, for instance, that with 32-bit integers you shouldn't use more than ~50000 values before a statistician could call you out with a better than 99% level of confidence. The solution, used by many modern PRNGs, is to have a larger state space and collapse it down to output a 32- or 64-bit result. Since multiple states can produce the same output, duplicates will occur in the output stream without the entire stream being replicated. It looks like that's what PyTorch is doing.
Given the larger state space, why allow seeding with a single integer? Convenience. For instance, Mersenne Twister has a 19,937 bit state space, but most people don't want to enter that much info to kick-start it. You can if you want to, but most people use the front-end which populates the full state space from a single integer input.
answered Nov 23 '18 at 23:21
pjspjs
13k41440
13k41440
add a comment |
add a comment |
Thanks for contributing an answer to Stack Overflow!
- Please be sure to answer the question. Provide details and share your research!
But avoid …
- Asking for help, clarification, or responding to other answers.
- Making statements based on opinion; back them up with references or personal experience.
To learn more, see our tips on writing great answers.
Sign up or log in
StackExchange.ready(function () {
StackExchange.helpers.onClickDraftSave('#login-link');
});
Sign up using Google
Sign up using Facebook
Sign up using Email and Password
Post as a guest
Required, but never shown
StackExchange.ready(
function () {
StackExchange.openid.initPostLogin('.new-post-login', 'https%3a%2f%2fstackoverflow.com%2fquestions%2f53452049%2funderstanding-seed-of-a-bytetensor-in-pytorch%23new-answer', 'question_page');
}
);
Post as a guest
Required, but never shown
Sign up or log in
StackExchange.ready(function () {
StackExchange.helpers.onClickDraftSave('#login-link');
});
Sign up using Google
Sign up using Facebook
Sign up using Email and Password
Post as a guest
Required, but never shown
Sign up or log in
StackExchange.ready(function () {
StackExchange.helpers.onClickDraftSave('#login-link');
});
Sign up using Google
Sign up using Facebook
Sign up using Email and Password
Post as a guest
Required, but never shown
Sign up or log in
StackExchange.ready(function () {
StackExchange.helpers.onClickDraftSave('#login-link');
});
Sign up using Google
Sign up using Facebook
Sign up using Email and Password
Sign up using Google
Sign up using Facebook
Sign up using Email and Password
Post as a guest
Required, but never shown
Required, but never shown
Required, but never shown
Required, but never shown
Required, but never shown
Required, but never shown
Required, but never shown
Required, but never shown
Required, but never shown
k7UEI,1rG0