Training vs validation set
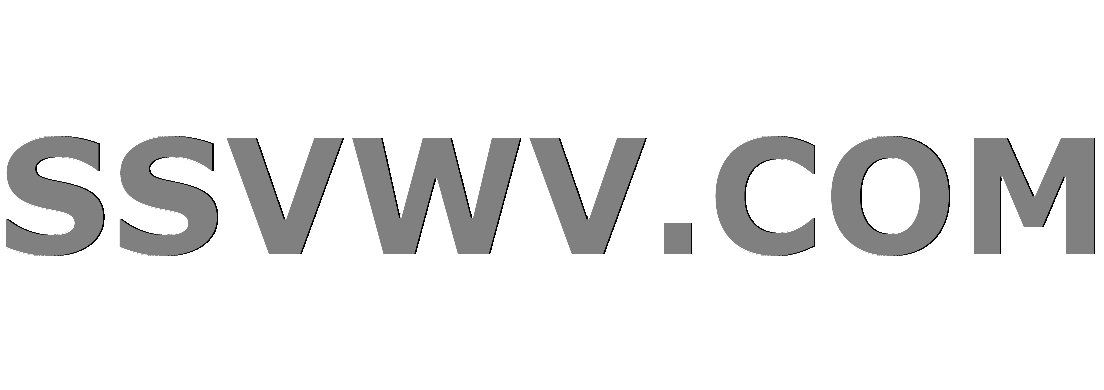
Multi tool use
$begingroup$
I have a data set with which I am trying to find correlations.
I split the data into a training set and a validation set. I also have a solver I built which finds the "best coefficients" to give me the best results on the training set.
After solving for the training set, the validation set shows completely different results which do not support the results on the training set.
I then made my solver output all of the results, as opposed to only the best results. There are cases which show positive, very similar results in both the training and validation sets, and there are results that show different results in both sets.
Is it okay to choose, by hand, the results that show the most similar and most positive results in both validation and training sets, or does this defeat the purpose of the validation set and invalidate the results?
data-analysis
$endgroup$
add a comment |
$begingroup$
I have a data set with which I am trying to find correlations.
I split the data into a training set and a validation set. I also have a solver I built which finds the "best coefficients" to give me the best results on the training set.
After solving for the training set, the validation set shows completely different results which do not support the results on the training set.
I then made my solver output all of the results, as opposed to only the best results. There are cases which show positive, very similar results in both the training and validation sets, and there are results that show different results in both sets.
Is it okay to choose, by hand, the results that show the most similar and most positive results in both validation and training sets, or does this defeat the purpose of the validation set and invalidate the results?
data-analysis
$endgroup$
1
$begingroup$
Maybe move to Data Science?
$endgroup$
– Paul Childs
Dec 10 '18 at 7:45
$begingroup$
Your best bet here is to use cross-validation, if you want to improve the performance of your model on the validation set. As indicated elsewhere, choosing the best results by hand is a BIG no-no, I would agree.
$endgroup$
– Adrian Keister
Dec 10 '18 at 14:57
add a comment |
$begingroup$
I have a data set with which I am trying to find correlations.
I split the data into a training set and a validation set. I also have a solver I built which finds the "best coefficients" to give me the best results on the training set.
After solving for the training set, the validation set shows completely different results which do not support the results on the training set.
I then made my solver output all of the results, as opposed to only the best results. There are cases which show positive, very similar results in both the training and validation sets, and there are results that show different results in both sets.
Is it okay to choose, by hand, the results that show the most similar and most positive results in both validation and training sets, or does this defeat the purpose of the validation set and invalidate the results?
data-analysis
$endgroup$
I have a data set with which I am trying to find correlations.
I split the data into a training set and a validation set. I also have a solver I built which finds the "best coefficients" to give me the best results on the training set.
After solving for the training set, the validation set shows completely different results which do not support the results on the training set.
I then made my solver output all of the results, as opposed to only the best results. There are cases which show positive, very similar results in both the training and validation sets, and there are results that show different results in both sets.
Is it okay to choose, by hand, the results that show the most similar and most positive results in both validation and training sets, or does this defeat the purpose of the validation set and invalidate the results?
data-analysis
data-analysis
asked Dec 10 '18 at 7:26
FrankFrank
16210
16210
1
$begingroup$
Maybe move to Data Science?
$endgroup$
– Paul Childs
Dec 10 '18 at 7:45
$begingroup$
Your best bet here is to use cross-validation, if you want to improve the performance of your model on the validation set. As indicated elsewhere, choosing the best results by hand is a BIG no-no, I would agree.
$endgroup$
– Adrian Keister
Dec 10 '18 at 14:57
add a comment |
1
$begingroup$
Maybe move to Data Science?
$endgroup$
– Paul Childs
Dec 10 '18 at 7:45
$begingroup$
Your best bet here is to use cross-validation, if you want to improve the performance of your model on the validation set. As indicated elsewhere, choosing the best results by hand is a BIG no-no, I would agree.
$endgroup$
– Adrian Keister
Dec 10 '18 at 14:57
1
1
$begingroup$
Maybe move to Data Science?
$endgroup$
– Paul Childs
Dec 10 '18 at 7:45
$begingroup$
Maybe move to Data Science?
$endgroup$
– Paul Childs
Dec 10 '18 at 7:45
$begingroup$
Your best bet here is to use cross-validation, if you want to improve the performance of your model on the validation set. As indicated elsewhere, choosing the best results by hand is a BIG no-no, I would agree.
$endgroup$
– Adrian Keister
Dec 10 '18 at 14:57
$begingroup$
Your best bet here is to use cross-validation, if you want to improve the performance of your model on the validation set. As indicated elsewhere, choosing the best results by hand is a BIG no-no, I would agree.
$endgroup$
– Adrian Keister
Dec 10 '18 at 14:57
add a comment |
1 Answer
1
active
oldest
votes
$begingroup$
This absolutely will invalidate the results. Data science is meant to be scientific; free from bias. It's ok to make a hypothesis and test it out, but if the results aren't what you want, that's ok, you revise the hypothesis and try again. That's what good science does. But manual "data wrangling" is a big no-no.
$endgroup$
$begingroup$
Thanks for your answer. I worry that my solver is just finding outliers in the data, and focusing on them. The results that match both might be the third or fourth best results that the solver found. It is still technically found by the solver. Does this make any difference?
$endgroup$
– Frank
Dec 10 '18 at 7:55
$begingroup$
A least mean squares regression for example is biased towards outliers, due to the nonlinearity of the square. There are other means - biased differently - as well as techniques for filtering out noise, but these shouldn't be done by hand, but based on a knowledge of the expected error.
$endgroup$
– Paul Childs
Dec 10 '18 at 23:22
add a comment |
Your Answer
StackExchange.ifUsing("editor", function () {
return StackExchange.using("mathjaxEditing", function () {
StackExchange.MarkdownEditor.creationCallbacks.add(function (editor, postfix) {
StackExchange.mathjaxEditing.prepareWmdForMathJax(editor, postfix, [["$", "$"], ["\\(","\\)"]]);
});
});
}, "mathjax-editing");
StackExchange.ready(function() {
var channelOptions = {
tags: "".split(" "),
id: "69"
};
initTagRenderer("".split(" "), "".split(" "), channelOptions);
StackExchange.using("externalEditor", function() {
// Have to fire editor after snippets, if snippets enabled
if (StackExchange.settings.snippets.snippetsEnabled) {
StackExchange.using("snippets", function() {
createEditor();
});
}
else {
createEditor();
}
});
function createEditor() {
StackExchange.prepareEditor({
heartbeatType: 'answer',
autoActivateHeartbeat: false,
convertImagesToLinks: true,
noModals: true,
showLowRepImageUploadWarning: true,
reputationToPostImages: 10,
bindNavPrevention: true,
postfix: "",
imageUploader: {
brandingHtml: "Powered by u003ca class="icon-imgur-white" href="https://imgur.com/"u003eu003c/au003e",
contentPolicyHtml: "User contributions licensed under u003ca href="https://creativecommons.org/licenses/by-sa/3.0/"u003ecc by-sa 3.0 with attribution requiredu003c/au003e u003ca href="https://stackoverflow.com/legal/content-policy"u003e(content policy)u003c/au003e",
allowUrls: true
},
noCode: true, onDemand: true,
discardSelector: ".discard-answer"
,immediatelyShowMarkdownHelp:true
});
}
});
Sign up or log in
StackExchange.ready(function () {
StackExchange.helpers.onClickDraftSave('#login-link');
});
Sign up using Google
Sign up using Facebook
Sign up using Email and Password
Post as a guest
Required, but never shown
StackExchange.ready(
function () {
StackExchange.openid.initPostLogin('.new-post-login', 'https%3a%2f%2fmath.stackexchange.com%2fquestions%2f3033582%2ftraining-vs-validation-set%23new-answer', 'question_page');
}
);
Post as a guest
Required, but never shown
1 Answer
1
active
oldest
votes
1 Answer
1
active
oldest
votes
active
oldest
votes
active
oldest
votes
$begingroup$
This absolutely will invalidate the results. Data science is meant to be scientific; free from bias. It's ok to make a hypothesis and test it out, but if the results aren't what you want, that's ok, you revise the hypothesis and try again. That's what good science does. But manual "data wrangling" is a big no-no.
$endgroup$
$begingroup$
Thanks for your answer. I worry that my solver is just finding outliers in the data, and focusing on them. The results that match both might be the third or fourth best results that the solver found. It is still technically found by the solver. Does this make any difference?
$endgroup$
– Frank
Dec 10 '18 at 7:55
$begingroup$
A least mean squares regression for example is biased towards outliers, due to the nonlinearity of the square. There are other means - biased differently - as well as techniques for filtering out noise, but these shouldn't be done by hand, but based on a knowledge of the expected error.
$endgroup$
– Paul Childs
Dec 10 '18 at 23:22
add a comment |
$begingroup$
This absolutely will invalidate the results. Data science is meant to be scientific; free from bias. It's ok to make a hypothesis and test it out, but if the results aren't what you want, that's ok, you revise the hypothesis and try again. That's what good science does. But manual "data wrangling" is a big no-no.
$endgroup$
$begingroup$
Thanks for your answer. I worry that my solver is just finding outliers in the data, and focusing on them. The results that match both might be the third or fourth best results that the solver found. It is still technically found by the solver. Does this make any difference?
$endgroup$
– Frank
Dec 10 '18 at 7:55
$begingroup$
A least mean squares regression for example is biased towards outliers, due to the nonlinearity of the square. There are other means - biased differently - as well as techniques for filtering out noise, but these shouldn't be done by hand, but based on a knowledge of the expected error.
$endgroup$
– Paul Childs
Dec 10 '18 at 23:22
add a comment |
$begingroup$
This absolutely will invalidate the results. Data science is meant to be scientific; free from bias. It's ok to make a hypothesis and test it out, but if the results aren't what you want, that's ok, you revise the hypothesis and try again. That's what good science does. But manual "data wrangling" is a big no-no.
$endgroup$
This absolutely will invalidate the results. Data science is meant to be scientific; free from bias. It's ok to make a hypothesis and test it out, but if the results aren't what you want, that's ok, you revise the hypothesis and try again. That's what good science does. But manual "data wrangling" is a big no-no.
answered Dec 10 '18 at 7:44


Paul ChildsPaul Childs
1757
1757
$begingroup$
Thanks for your answer. I worry that my solver is just finding outliers in the data, and focusing on them. The results that match both might be the third or fourth best results that the solver found. It is still technically found by the solver. Does this make any difference?
$endgroup$
– Frank
Dec 10 '18 at 7:55
$begingroup$
A least mean squares regression for example is biased towards outliers, due to the nonlinearity of the square. There are other means - biased differently - as well as techniques for filtering out noise, but these shouldn't be done by hand, but based on a knowledge of the expected error.
$endgroup$
– Paul Childs
Dec 10 '18 at 23:22
add a comment |
$begingroup$
Thanks for your answer. I worry that my solver is just finding outliers in the data, and focusing on them. The results that match both might be the third or fourth best results that the solver found. It is still technically found by the solver. Does this make any difference?
$endgroup$
– Frank
Dec 10 '18 at 7:55
$begingroup$
A least mean squares regression for example is biased towards outliers, due to the nonlinearity of the square. There are other means - biased differently - as well as techniques for filtering out noise, but these shouldn't be done by hand, but based on a knowledge of the expected error.
$endgroup$
– Paul Childs
Dec 10 '18 at 23:22
$begingroup$
Thanks for your answer. I worry that my solver is just finding outliers in the data, and focusing on them. The results that match both might be the third or fourth best results that the solver found. It is still technically found by the solver. Does this make any difference?
$endgroup$
– Frank
Dec 10 '18 at 7:55
$begingroup$
Thanks for your answer. I worry that my solver is just finding outliers in the data, and focusing on them. The results that match both might be the third or fourth best results that the solver found. It is still technically found by the solver. Does this make any difference?
$endgroup$
– Frank
Dec 10 '18 at 7:55
$begingroup$
A least mean squares regression for example is biased towards outliers, due to the nonlinearity of the square. There are other means - biased differently - as well as techniques for filtering out noise, but these shouldn't be done by hand, but based on a knowledge of the expected error.
$endgroup$
– Paul Childs
Dec 10 '18 at 23:22
$begingroup$
A least mean squares regression for example is biased towards outliers, due to the nonlinearity of the square. There are other means - biased differently - as well as techniques for filtering out noise, but these shouldn't be done by hand, but based on a knowledge of the expected error.
$endgroup$
– Paul Childs
Dec 10 '18 at 23:22
add a comment |
Thanks for contributing an answer to Mathematics Stack Exchange!
- Please be sure to answer the question. Provide details and share your research!
But avoid …
- Asking for help, clarification, or responding to other answers.
- Making statements based on opinion; back them up with references or personal experience.
Use MathJax to format equations. MathJax reference.
To learn more, see our tips on writing great answers.
Sign up or log in
StackExchange.ready(function () {
StackExchange.helpers.onClickDraftSave('#login-link');
});
Sign up using Google
Sign up using Facebook
Sign up using Email and Password
Post as a guest
Required, but never shown
StackExchange.ready(
function () {
StackExchange.openid.initPostLogin('.new-post-login', 'https%3a%2f%2fmath.stackexchange.com%2fquestions%2f3033582%2ftraining-vs-validation-set%23new-answer', 'question_page');
}
);
Post as a guest
Required, but never shown
Sign up or log in
StackExchange.ready(function () {
StackExchange.helpers.onClickDraftSave('#login-link');
});
Sign up using Google
Sign up using Facebook
Sign up using Email and Password
Post as a guest
Required, but never shown
Sign up or log in
StackExchange.ready(function () {
StackExchange.helpers.onClickDraftSave('#login-link');
});
Sign up using Google
Sign up using Facebook
Sign up using Email and Password
Post as a guest
Required, but never shown
Sign up or log in
StackExchange.ready(function () {
StackExchange.helpers.onClickDraftSave('#login-link');
});
Sign up using Google
Sign up using Facebook
Sign up using Email and Password
Sign up using Google
Sign up using Facebook
Sign up using Email and Password
Post as a guest
Required, but never shown
Required, but never shown
Required, but never shown
Required, but never shown
Required, but never shown
Required, but never shown
Required, but never shown
Required, but never shown
Required, but never shown
TS2E9ICQP,FFmgHjFY6qaK7,G
1
$begingroup$
Maybe move to Data Science?
$endgroup$
– Paul Childs
Dec 10 '18 at 7:45
$begingroup$
Your best bet here is to use cross-validation, if you want to improve the performance of your model on the validation set. As indicated elsewhere, choosing the best results by hand is a BIG no-no, I would agree.
$endgroup$
– Adrian Keister
Dec 10 '18 at 14:57