Input contains Nan with Tfidf vectorizer output
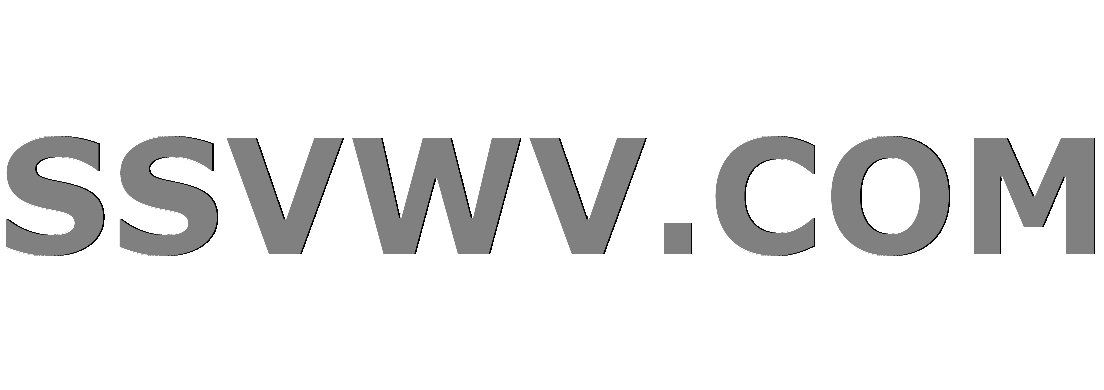
Multi tool use
up vote
1
down vote
favorite
I've got a problem with the output of Tfidf Vectorizer and I've test many solutions given in other topics and nothing works.
I have a csv with two columns : one column test containing ... text and a column score.
And I want to be able to predict a new score based on a text I will be able to input.
I think the better solution is to use a linear regression based on tfidf analys on the text.
My code is the following :
datas = pandas.read_csv('Data/gucci-account-
prediction.csv',delimiter=';')
datas['score'] = datas['retweets'] + datas['likes']
import re
def tokenizer(text):
if text:
result = re.findall('[a-z]{2,}', text.lower())
else:
result =
return result
X = datas['text'].values
y = datas['score'].values
vect = TfidfVectorizer(tokenizer=tokenizer,stop_words='english',dtype=np.float32)
X_train = vect.fit_transform(X)
lr = Ridge(alpha=1.0)
lr.fit(X_train,y)
And I have the following error : Input contains NaN, infinity or a value too large for dtype('float64').
I already verified and my dataframe ( before vectorization ) contains no nan value so I don't understand why my X matrix would contain any nan or infinite value.
Would you have a solution so it works ? Thank you
python regression vectorization
add a comment |
up vote
1
down vote
favorite
I've got a problem with the output of Tfidf Vectorizer and I've test many solutions given in other topics and nothing works.
I have a csv with two columns : one column test containing ... text and a column score.
And I want to be able to predict a new score based on a text I will be able to input.
I think the better solution is to use a linear regression based on tfidf analys on the text.
My code is the following :
datas = pandas.read_csv('Data/gucci-account-
prediction.csv',delimiter=';')
datas['score'] = datas['retweets'] + datas['likes']
import re
def tokenizer(text):
if text:
result = re.findall('[a-z]{2,}', text.lower())
else:
result =
return result
X = datas['text'].values
y = datas['score'].values
vect = TfidfVectorizer(tokenizer=tokenizer,stop_words='english',dtype=np.float32)
X_train = vect.fit_transform(X)
lr = Ridge(alpha=1.0)
lr.fit(X_train,y)
And I have the following error : Input contains NaN, infinity or a value too large for dtype('float64').
I already verified and my dataframe ( before vectorization ) contains no nan value so I don't understand why my X matrix would contain any nan or infinite value.
Would you have a solution so it works ? Thank you
python regression vectorization
Please, add more information on the stack track (which line rises the error would be useful)
– Julian Peller
Nov 21 at 13:05
add a comment |
up vote
1
down vote
favorite
up vote
1
down vote
favorite
I've got a problem with the output of Tfidf Vectorizer and I've test many solutions given in other topics and nothing works.
I have a csv with two columns : one column test containing ... text and a column score.
And I want to be able to predict a new score based on a text I will be able to input.
I think the better solution is to use a linear regression based on tfidf analys on the text.
My code is the following :
datas = pandas.read_csv('Data/gucci-account-
prediction.csv',delimiter=';')
datas['score'] = datas['retweets'] + datas['likes']
import re
def tokenizer(text):
if text:
result = re.findall('[a-z]{2,}', text.lower())
else:
result =
return result
X = datas['text'].values
y = datas['score'].values
vect = TfidfVectorizer(tokenizer=tokenizer,stop_words='english',dtype=np.float32)
X_train = vect.fit_transform(X)
lr = Ridge(alpha=1.0)
lr.fit(X_train,y)
And I have the following error : Input contains NaN, infinity or a value too large for dtype('float64').
I already verified and my dataframe ( before vectorization ) contains no nan value so I don't understand why my X matrix would contain any nan or infinite value.
Would you have a solution so it works ? Thank you
python regression vectorization
I've got a problem with the output of Tfidf Vectorizer and I've test many solutions given in other topics and nothing works.
I have a csv with two columns : one column test containing ... text and a column score.
And I want to be able to predict a new score based on a text I will be able to input.
I think the better solution is to use a linear regression based on tfidf analys on the text.
My code is the following :
datas = pandas.read_csv('Data/gucci-account-
prediction.csv',delimiter=';')
datas['score'] = datas['retweets'] + datas['likes']
import re
def tokenizer(text):
if text:
result = re.findall('[a-z]{2,}', text.lower())
else:
result =
return result
X = datas['text'].values
y = datas['score'].values
vect = TfidfVectorizer(tokenizer=tokenizer,stop_words='english',dtype=np.float32)
X_train = vect.fit_transform(X)
lr = Ridge(alpha=1.0)
lr.fit(X_train,y)
And I have the following error : Input contains NaN, infinity or a value too large for dtype('float64').
I already verified and my dataframe ( before vectorization ) contains no nan value so I don't understand why my X matrix would contain any nan or infinite value.
Would you have a solution so it works ? Thank you
python regression vectorization
python regression vectorization
asked Nov 21 at 10:24
Pierre Ftn
2615
2615
Please, add more information on the stack track (which line rises the error would be useful)
– Julian Peller
Nov 21 at 13:05
add a comment |
Please, add more information on the stack track (which line rises the error would be useful)
– Julian Peller
Nov 21 at 13:05
Please, add more information on the stack track (which line rises the error would be useful)
– Julian Peller
Nov 21 at 13:05
Please, add more information on the stack track (which line rises the error would be useful)
– Julian Peller
Nov 21 at 13:05
add a comment |
active
oldest
votes
active
oldest
votes
active
oldest
votes
active
oldest
votes
active
oldest
votes
Thanks for contributing an answer to Stack Overflow!
- Please be sure to answer the question. Provide details and share your research!
But avoid …
- Asking for help, clarification, or responding to other answers.
- Making statements based on opinion; back them up with references or personal experience.
To learn more, see our tips on writing great answers.
Some of your past answers have not been well-received, and you're in danger of being blocked from answering.
Please pay close attention to the following guidance:
- Please be sure to answer the question. Provide details and share your research!
But avoid …
- Asking for help, clarification, or responding to other answers.
- Making statements based on opinion; back them up with references or personal experience.
To learn more, see our tips on writing great answers.
Sign up or log in
StackExchange.ready(function () {
StackExchange.helpers.onClickDraftSave('#login-link');
});
Sign up using Google
Sign up using Facebook
Sign up using Email and Password
Post as a guest
Required, but never shown
StackExchange.ready(
function () {
StackExchange.openid.initPostLogin('.new-post-login', 'https%3a%2f%2fstackoverflow.com%2fquestions%2f53409968%2finput-contains-nan-with-tfidf-vectorizer-output%23new-answer', 'question_page');
}
);
Post as a guest
Required, but never shown
Sign up or log in
StackExchange.ready(function () {
StackExchange.helpers.onClickDraftSave('#login-link');
});
Sign up using Google
Sign up using Facebook
Sign up using Email and Password
Post as a guest
Required, but never shown
Sign up or log in
StackExchange.ready(function () {
StackExchange.helpers.onClickDraftSave('#login-link');
});
Sign up using Google
Sign up using Facebook
Sign up using Email and Password
Post as a guest
Required, but never shown
Sign up or log in
StackExchange.ready(function () {
StackExchange.helpers.onClickDraftSave('#login-link');
});
Sign up using Google
Sign up using Facebook
Sign up using Email and Password
Sign up using Google
Sign up using Facebook
Sign up using Email and Password
Post as a guest
Required, but never shown
Required, but never shown
Required, but never shown
Required, but never shown
Required, but never shown
Required, but never shown
Required, but never shown
Required, but never shown
Required, but never shown
3Y,QaOHDH29jgO9j,iBgRrvckF7mk6fv1WYAhqHuHhPw0J4vy XOXLC0LzcqFe6n o2unWtSWknWUNZUoL9lbE5b m0 YP
Please, add more information on the stack track (which line rises the error would be useful)
– Julian Peller
Nov 21 at 13:05