Why am I getting a score of 0.0 when finding the score of test data using Gaussian NB classifier?
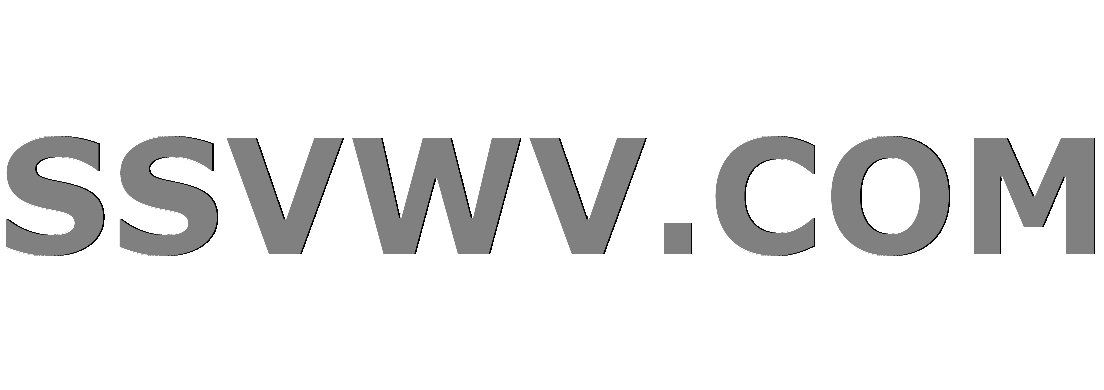
Multi tool use
up vote
0
down vote
favorite
I have two different data sets. One for training my classifier and the other one is for testing. Both the datasets are text files with two columns separated by a ",". FIrst column (numbers) is for the independent variable (group) and the second column is for the dependent variable.
Training data set
(just few lines for example. there are no empty lines between each row):
EMI3776438,1
EMI3776438,1
EMI3669492,1
EMI3752004,1
Testing data setup
(as you can see, i have picked data from the training data to be sure that the score surely can't be zero)
EMI3776438,1
Code in Python 3.6:
# #all the import statements have been ignored to keep the code short
# #loading the training data set
training_file_path=r'C:UsersyyyDesktopmy filespythonMachine learningCarepackmodified_columns.txt'
from sklearn import preprocessing
le = preprocessing.LabelEncoder()
training_file_data = pandas.read_table(training_file_path,
header=None,
names=['numbers','group'],
sep=',')
training_file_data = training_file_data.apply(le.fit_transform)
features = ['numbers']
x = training_file_data[features]
y = training_file_data["group"]
from sklearn.model_selection import train_test_split
training_x,testing_x, training_y, testing_y = train_test_split(x, y,
random_state=0,
test_size=0.1)
from sklearn.naive_bayes import GaussianNB
gnb= GaussianNB()
gnb.fit(training_x, training_y)
# #loading the testing data
testing_final_path=r"C:UsersyyyDesktopmy filespythonMachine learningCarepacktesting_final.txt"
testing_sample_data=pandas.read_table(testing_final_path,
sep=',',
header=None,
names=['numbers','group'])
testing_sample_data = testing_sample_data.apply(le.fit_transform)
category = ["numbers"]
testing_sample_data_x = testing_sample_data[category]
# #finding the score of the test data
print(gnb.score(testing_sample_data_x, testing_sample_data["group"]))
python-3.x pandas machine-learning scikit-learn gaussian
add a comment |
up vote
0
down vote
favorite
I have two different data sets. One for training my classifier and the other one is for testing. Both the datasets are text files with two columns separated by a ",". FIrst column (numbers) is for the independent variable (group) and the second column is for the dependent variable.
Training data set
(just few lines for example. there are no empty lines between each row):
EMI3776438,1
EMI3776438,1
EMI3669492,1
EMI3752004,1
Testing data setup
(as you can see, i have picked data from the training data to be sure that the score surely can't be zero)
EMI3776438,1
Code in Python 3.6:
# #all the import statements have been ignored to keep the code short
# #loading the training data set
training_file_path=r'C:UsersyyyDesktopmy filespythonMachine learningCarepackmodified_columns.txt'
from sklearn import preprocessing
le = preprocessing.LabelEncoder()
training_file_data = pandas.read_table(training_file_path,
header=None,
names=['numbers','group'],
sep=',')
training_file_data = training_file_data.apply(le.fit_transform)
features = ['numbers']
x = training_file_data[features]
y = training_file_data["group"]
from sklearn.model_selection import train_test_split
training_x,testing_x, training_y, testing_y = train_test_split(x, y,
random_state=0,
test_size=0.1)
from sklearn.naive_bayes import GaussianNB
gnb= GaussianNB()
gnb.fit(training_x, training_y)
# #loading the testing data
testing_final_path=r"C:UsersyyyDesktopmy filespythonMachine learningCarepacktesting_final.txt"
testing_sample_data=pandas.read_table(testing_final_path,
sep=',',
header=None,
names=['numbers','group'])
testing_sample_data = testing_sample_data.apply(le.fit_transform)
category = ["numbers"]
testing_sample_data_x = testing_sample_data[category]
# #finding the score of the test data
print(gnb.score(testing_sample_data_x, testing_sample_data["group"]))
python-3.x pandas machine-learning scikit-learn gaussian
add a comment |
up vote
0
down vote
favorite
up vote
0
down vote
favorite
I have two different data sets. One for training my classifier and the other one is for testing. Both the datasets are text files with two columns separated by a ",". FIrst column (numbers) is for the independent variable (group) and the second column is for the dependent variable.
Training data set
(just few lines for example. there are no empty lines between each row):
EMI3776438,1
EMI3776438,1
EMI3669492,1
EMI3752004,1
Testing data setup
(as you can see, i have picked data from the training data to be sure that the score surely can't be zero)
EMI3776438,1
Code in Python 3.6:
# #all the import statements have been ignored to keep the code short
# #loading the training data set
training_file_path=r'C:UsersyyyDesktopmy filespythonMachine learningCarepackmodified_columns.txt'
from sklearn import preprocessing
le = preprocessing.LabelEncoder()
training_file_data = pandas.read_table(training_file_path,
header=None,
names=['numbers','group'],
sep=',')
training_file_data = training_file_data.apply(le.fit_transform)
features = ['numbers']
x = training_file_data[features]
y = training_file_data["group"]
from sklearn.model_selection import train_test_split
training_x,testing_x, training_y, testing_y = train_test_split(x, y,
random_state=0,
test_size=0.1)
from sklearn.naive_bayes import GaussianNB
gnb= GaussianNB()
gnb.fit(training_x, training_y)
# #loading the testing data
testing_final_path=r"C:UsersyyyDesktopmy filespythonMachine learningCarepacktesting_final.txt"
testing_sample_data=pandas.read_table(testing_final_path,
sep=',',
header=None,
names=['numbers','group'])
testing_sample_data = testing_sample_data.apply(le.fit_transform)
category = ["numbers"]
testing_sample_data_x = testing_sample_data[category]
# #finding the score of the test data
print(gnb.score(testing_sample_data_x, testing_sample_data["group"]))
python-3.x pandas machine-learning scikit-learn gaussian
I have two different data sets. One for training my classifier and the other one is for testing. Both the datasets are text files with two columns separated by a ",". FIrst column (numbers) is for the independent variable (group) and the second column is for the dependent variable.
Training data set
(just few lines for example. there are no empty lines between each row):
EMI3776438,1
EMI3776438,1
EMI3669492,1
EMI3752004,1
Testing data setup
(as you can see, i have picked data from the training data to be sure that the score surely can't be zero)
EMI3776438,1
Code in Python 3.6:
# #all the import statements have been ignored to keep the code short
# #loading the training data set
training_file_path=r'C:UsersyyyDesktopmy filespythonMachine learningCarepackmodified_columns.txt'
from sklearn import preprocessing
le = preprocessing.LabelEncoder()
training_file_data = pandas.read_table(training_file_path,
header=None,
names=['numbers','group'],
sep=',')
training_file_data = training_file_data.apply(le.fit_transform)
features = ['numbers']
x = training_file_data[features]
y = training_file_data["group"]
from sklearn.model_selection import train_test_split
training_x,testing_x, training_y, testing_y = train_test_split(x, y,
random_state=0,
test_size=0.1)
from sklearn.naive_bayes import GaussianNB
gnb= GaussianNB()
gnb.fit(training_x, training_y)
# #loading the testing data
testing_final_path=r"C:UsersyyyDesktopmy filespythonMachine learningCarepacktesting_final.txt"
testing_sample_data=pandas.read_table(testing_final_path,
sep=',',
header=None,
names=['numbers','group'])
testing_sample_data = testing_sample_data.apply(le.fit_transform)
category = ["numbers"]
testing_sample_data_x = testing_sample_data[category]
# #finding the score of the test data
print(gnb.score(testing_sample_data_x, testing_sample_data["group"]))
python-3.x pandas machine-learning scikit-learn gaussian
python-3.x pandas machine-learning scikit-learn gaussian
edited Nov 22 at 6:24
asked Nov 21 at 10:18
wanttomasterpython
909
909
add a comment |
add a comment |
1 Answer
1
active
oldest
votes
up vote
0
down vote
First, the above data samples dont show how many classes are there in it. You need to describe more about it.
Secondly, you are calling le.fit_transform
again on test data which will forget all the training samples mappings from strings to numbers. The LabelEncoder
le will start encoding the test data again from scratch, which will not be equal to how it mapped training data. So the input to GaussianNB
is now incorrect and hence incorrect results.
Change that to:
testing_sample_data = testing_sample_data.apply(le.transform)
UPDATE:
I'm sorry I overlooked the fact that you had two columns in your data. LabelEncoder
only works on a single column of data. For making it work on multiple pandas columns at once, look at the answers of following question:
- Label encoding across multiple columns in scikit-learn
If you are using the latest version of scikit (0.20
) or can update to it, then you would not need any such hacks and directly use the OrdinalEncoder
:
from sklearn.preprocessing import OrdinalEncoder
enc = OrdinalEncoder()
training_file_data = enc.fit_transform(training_file_data)
And during testing:
training_file_data = enc.transform(training_file_data)
I changed the line to what you had asked me to but there is a different error now. The error arises from the changed line. ValueError: ("y contains previously unseen labels: 'numbers'", 'occurred at index numbers')
– wanttomasterpython
Nov 22 at 6:28
add a comment |
1 Answer
1
active
oldest
votes
1 Answer
1
active
oldest
votes
active
oldest
votes
active
oldest
votes
up vote
0
down vote
First, the above data samples dont show how many classes are there in it. You need to describe more about it.
Secondly, you are calling le.fit_transform
again on test data which will forget all the training samples mappings from strings to numbers. The LabelEncoder
le will start encoding the test data again from scratch, which will not be equal to how it mapped training data. So the input to GaussianNB
is now incorrect and hence incorrect results.
Change that to:
testing_sample_data = testing_sample_data.apply(le.transform)
UPDATE:
I'm sorry I overlooked the fact that you had two columns in your data. LabelEncoder
only works on a single column of data. For making it work on multiple pandas columns at once, look at the answers of following question:
- Label encoding across multiple columns in scikit-learn
If you are using the latest version of scikit (0.20
) or can update to it, then you would not need any such hacks and directly use the OrdinalEncoder
:
from sklearn.preprocessing import OrdinalEncoder
enc = OrdinalEncoder()
training_file_data = enc.fit_transform(training_file_data)
And during testing:
training_file_data = enc.transform(training_file_data)
I changed the line to what you had asked me to but there is a different error now. The error arises from the changed line. ValueError: ("y contains previously unseen labels: 'numbers'", 'occurred at index numbers')
– wanttomasterpython
Nov 22 at 6:28
add a comment |
up vote
0
down vote
First, the above data samples dont show how many classes are there in it. You need to describe more about it.
Secondly, you are calling le.fit_transform
again on test data which will forget all the training samples mappings from strings to numbers. The LabelEncoder
le will start encoding the test data again from scratch, which will not be equal to how it mapped training data. So the input to GaussianNB
is now incorrect and hence incorrect results.
Change that to:
testing_sample_data = testing_sample_data.apply(le.transform)
UPDATE:
I'm sorry I overlooked the fact that you had two columns in your data. LabelEncoder
only works on a single column of data. For making it work on multiple pandas columns at once, look at the answers of following question:
- Label encoding across multiple columns in scikit-learn
If you are using the latest version of scikit (0.20
) or can update to it, then you would not need any such hacks and directly use the OrdinalEncoder
:
from sklearn.preprocessing import OrdinalEncoder
enc = OrdinalEncoder()
training_file_data = enc.fit_transform(training_file_data)
And during testing:
training_file_data = enc.transform(training_file_data)
I changed the line to what you had asked me to but there is a different error now. The error arises from the changed line. ValueError: ("y contains previously unseen labels: 'numbers'", 'occurred at index numbers')
– wanttomasterpython
Nov 22 at 6:28
add a comment |
up vote
0
down vote
up vote
0
down vote
First, the above data samples dont show how many classes are there in it. You need to describe more about it.
Secondly, you are calling le.fit_transform
again on test data which will forget all the training samples mappings from strings to numbers. The LabelEncoder
le will start encoding the test data again from scratch, which will not be equal to how it mapped training data. So the input to GaussianNB
is now incorrect and hence incorrect results.
Change that to:
testing_sample_data = testing_sample_data.apply(le.transform)
UPDATE:
I'm sorry I overlooked the fact that you had two columns in your data. LabelEncoder
only works on a single column of data. For making it work on multiple pandas columns at once, look at the answers of following question:
- Label encoding across multiple columns in scikit-learn
If you are using the latest version of scikit (0.20
) or can update to it, then you would not need any such hacks and directly use the OrdinalEncoder
:
from sklearn.preprocessing import OrdinalEncoder
enc = OrdinalEncoder()
training_file_data = enc.fit_transform(training_file_data)
And during testing:
training_file_data = enc.transform(training_file_data)
First, the above data samples dont show how many classes are there in it. You need to describe more about it.
Secondly, you are calling le.fit_transform
again on test data which will forget all the training samples mappings from strings to numbers. The LabelEncoder
le will start encoding the test data again from scratch, which will not be equal to how it mapped training data. So the input to GaussianNB
is now incorrect and hence incorrect results.
Change that to:
testing_sample_data = testing_sample_data.apply(le.transform)
UPDATE:
I'm sorry I overlooked the fact that you had two columns in your data. LabelEncoder
only works on a single column of data. For making it work on multiple pandas columns at once, look at the answers of following question:
- Label encoding across multiple columns in scikit-learn
If you are using the latest version of scikit (0.20
) or can update to it, then you would not need any such hacks and directly use the OrdinalEncoder
:
from sklearn.preprocessing import OrdinalEncoder
enc = OrdinalEncoder()
training_file_data = enc.fit_transform(training_file_data)
And during testing:
training_file_data = enc.transform(training_file_data)
edited Nov 22 at 7:03
answered Nov 21 at 11:00
Vivek Kumar
14.3k41849
14.3k41849
I changed the line to what you had asked me to but there is a different error now. The error arises from the changed line. ValueError: ("y contains previously unseen labels: 'numbers'", 'occurred at index numbers')
– wanttomasterpython
Nov 22 at 6:28
add a comment |
I changed the line to what you had asked me to but there is a different error now. The error arises from the changed line. ValueError: ("y contains previously unseen labels: 'numbers'", 'occurred at index numbers')
– wanttomasterpython
Nov 22 at 6:28
I changed the line to what you had asked me to but there is a different error now. The error arises from the changed line. ValueError: ("y contains previously unseen labels: 'numbers'", 'occurred at index numbers')
– wanttomasterpython
Nov 22 at 6:28
I changed the line to what you had asked me to but there is a different error now. The error arises from the changed line. ValueError: ("y contains previously unseen labels: 'numbers'", 'occurred at index numbers')
– wanttomasterpython
Nov 22 at 6:28
add a comment |
Thanks for contributing an answer to Stack Overflow!
- Please be sure to answer the question. Provide details and share your research!
But avoid …
- Asking for help, clarification, or responding to other answers.
- Making statements based on opinion; back them up with references or personal experience.
To learn more, see our tips on writing great answers.
Some of your past answers have not been well-received, and you're in danger of being blocked from answering.
Please pay close attention to the following guidance:
- Please be sure to answer the question. Provide details and share your research!
But avoid …
- Asking for help, clarification, or responding to other answers.
- Making statements based on opinion; back them up with references or personal experience.
To learn more, see our tips on writing great answers.
Sign up or log in
StackExchange.ready(function () {
StackExchange.helpers.onClickDraftSave('#login-link');
});
Sign up using Google
Sign up using Facebook
Sign up using Email and Password
Post as a guest
Required, but never shown
StackExchange.ready(
function () {
StackExchange.openid.initPostLogin('.new-post-login', 'https%3a%2f%2fstackoverflow.com%2fquestions%2f53409843%2fwhy-am-i-getting-a-score-of-0-0-when-finding-the-score-of-test-data-using-gaussi%23new-answer', 'question_page');
}
);
Post as a guest
Required, but never shown
Sign up or log in
StackExchange.ready(function () {
StackExchange.helpers.onClickDraftSave('#login-link');
});
Sign up using Google
Sign up using Facebook
Sign up using Email and Password
Post as a guest
Required, but never shown
Sign up or log in
StackExchange.ready(function () {
StackExchange.helpers.onClickDraftSave('#login-link');
});
Sign up using Google
Sign up using Facebook
Sign up using Email and Password
Post as a guest
Required, but never shown
Sign up or log in
StackExchange.ready(function () {
StackExchange.helpers.onClickDraftSave('#login-link');
});
Sign up using Google
Sign up using Facebook
Sign up using Email and Password
Sign up using Google
Sign up using Facebook
Sign up using Email and Password
Post as a guest
Required, but never shown
Required, but never shown
Required, but never shown
Required, but never shown
Required, but never shown
Required, but never shown
Required, but never shown
Required, but never shown
Required, but never shown
0oOtH,R8Yj NU54sl,VzpogTL,S ZuPEuBOi5BbyIJlJyT,t6UXEq,Zir1at01z6ZCfoO adV,H1bKB0t4 LEbeIVVfLKmBWGvat53JQW1K